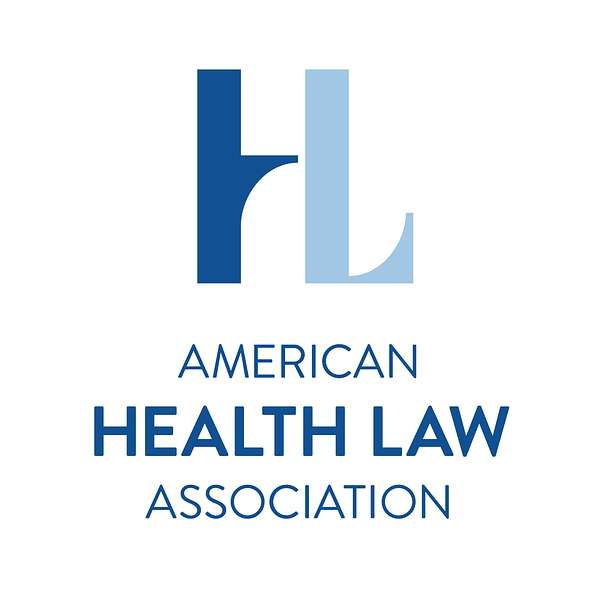
AHLA's Speaking of Health Law
The American Health Law Association (AHLA) is the largest nonprofit, nonpartisan educational organization devoted to legal issues in the health care field. AHLA's Speaking of Health Law podcasts offer thoughtful analysis and insightful commentary on the legal and policy issues affecting the American health care system.
AHLA's Speaking of Health Law
Fraud and Abuse: AI and Machine Learning—How Can Innovative Technology Help in the Fight Against Health Care Fraud and Abuse
In this episode of AHLA's monthly series on fraud and abuse issues, Matthew Wetzel, Associate General Counsel, Compliance Officer, GRAIL, speaks with Craig Freeman and Jerry Lewandowski, Berkeley Research Group (BRG), about artificial intelligence in health care. The podcast discusses how innovative tools can be used to root out compliance issues. From AHLA's Fraud and Abuse Practice Group. Sponsored by BRG.
Essential Legal Updates, Now in Audio
AHLA's popular Health Law Daily email newsletter is now a daily podcast, exclusively for AHLA Premium members. Get all your health law news from the major media outlets on this podcast! To subscribe and add this private podcast feed to your podcast app, go to americanhealthlaw.org/dailypodcast.
Stay At the Forefront of Health Legal Education
Learn more about AHLA and the educational resources available to the health law community at https://www.americanhealthlaw.org/.
The following message and support for A H L A is provided by Berkeley Research Group, a global consulting firm that helps organizations advance in the areas of disputes and investigations, corporate finance and strategy and operations. BRG helps clients stay ahead of what's next. For more information, visit think brg.com.
Speaker 2:Welcome to the American Health Law Associations Fraud and Abuse Podcast. I'm Matt Wetzel, chair of the ALA's Fraud Abuse Practice Group. Today we're talking about artificial intelligence and machine learning. How can innovative technological tools help in the fight against healthcare fraud and corruption? With me today are Craig Freeman and Jerry Lewandowski of B r g, who are experts in the field. Craig, Jerry, welcome.
Speaker 3:Hi, Matt. Good to be here.
Speaker 4:Yep. Thanks, Matt. Good to be here.
Speaker 2:Great to have you here today, guys, and, and, and we're really excited about the conversation. So, you know, let's jump right in. Tell us about your practice at B R G and how you got into the field of artificial intelligence and machine learning.
Speaker 3:Well, I'll, I'll go first. This is Craig. Uh, I am, uh, the practice lead for our artificial intelligence and machine learning practice. Uh, we had, uh, founded a company about four years ago that was purchased by B R G, uh, in this, uh, past year. And the whole goal is to bring more advanced technology tools to some of the traditional problems, uh, in, in including fraud and including compliance.
Speaker 4:And, and this is Jerry. I'm one of the leaders of our healthcare practice at B R G. And we've long had a practice that is, uh, heavy on data analytics in the healthcare space. And given the evolution of, um, some of the analytical techniques and what Craig's team team can do, there's such a natural fit for what a lot of our healthcare clients need from a fraud and abuse standpoint, from a, um, from a compliance standpoint, that we tend to work together quite a bit.
Speaker 2:That's great. And, and, and during our prep call for this episode, I just wanna let our listeners know, we dug into some technical topics, uh, and some really important, uh, areas for compliance and for legal issues in the healthcare space, whether you're in medical technology or, or whether you're in, uh, the provider area. But, uh, you know, uh, Craig, Jerry, I think maybe the best place to start is to level set a little bit what is artificial intelligence? What's machine learning? Uh, you talked about, um, supervised and unsupervised machine learning during our prep today, as well as collective intelligence. What are these concepts and what do we, what do our listeners, uh, the, uh, the A H L A, uh, uh, fraud and abuse practice group, uh, members, what do we need to know about these topics?
Speaker 3:Well, I don't think anyone's gonna become a data scientist, uh, on a half hour podcast today,<laugh>, but there were a, you listed some key concepts that it's really hard to get into the weeds on this stuff without understanding those concepts. Um, first off, artificial intelligence, I'm not sure it is anything. Um, we, we use a slide deck sometimes it shows, you know, 20 different components of, uh, artificial intelligence, whether that's machine learning, whether that's natural language processing, whether that's probiotics. And so what you have to do is you have to figure out within AI what, uh, area do you wanna work in? And what we do primarily is work in machine learning, which is where you give the, the, the, the computer system some level of data, and then it can go and improve itself. And usually that's an iterative process that you run again and again and again and again. Uh, supervised versus unsupervised learning are, um, are really important concepts. Supervised is where, uh, you take a training set and some lawyer, some analyst goes through the training set and labels, uh, the transactions or the documents as, as something, um, uh, whatever that is. Uh, and then, um, and then the system looks at those, uh, transactions and then creates a model to emulate that and goes forward unsupervised. You don't have that train set. The, uh, the, the, the, the main area in unsupervised learning that we try to focus on is called anomaly detection, which is that you build algorithms that go through the data and try to find transactions that aren't the same as the other transactions anomalous in some sort of way. Uh, it, and it could be a fraudulent transaction, it could be, uh, a false hit, but you go through, um, uh, the, the, uh, unsupervised without any training set of original material. And then lastly, uh, the, the, the idea of collective intelligence is the idea that the system is gonna be better if you have both data science expertise along with subject matter expertise and use both groups of people in the appropriate way. And you get a much better result than just using data scientists or just using domain experts. Jerry, do you have anything you wanted to add to that?
Speaker 4:No, I mean, the only thing I would echo is the collective intelligence piece. That, that we've really found that even when you're an expert in healthcare and even an expert in healthcare data, there are analytical techniques that are different with machine learning, where you can actually uncover things that we haven't been able to do historically with, um, more traditional techniques. So marrying the data scientists with the subject matter expertise is, is really valuable.
Speaker 2:I think you're hitting on a really, um, critical topic and, and, you know, how do, how do you respond to somebody who says, well, isn't this just, isn't machine learning just a computer doing the same keyword search that I would do, uh, as a lawyer or as a compliance professional, uh, when, when faced with a particular, uh, fraud and abuse issue?
Speaker 3:Well, it's, it's, it, it's not keyword searching at all. Uh, it, it, it's using various algorithms and you can test the algorithms to find out which one works best to, uh, to classify the in information. Uh, and it, it learns and improves, um, uh, o over time. And so one of the things, uh, that Matt, I think we talked about earlier was, uh, the idea that, uh, AI and, uh, machine learning in particular do a really good job at identifying privileged documents without doing keyword searches. Um, the, the, the, uh, the, uh, misunderstanding by many lawyers is that, uh, uh, everyone thinks that they write uniquely. And, and the fact of the matter is that lawyers write like lawyers, uh, they use a different number of syllables, uh, in their words, they use different length of sentences, they use footnotes, they use Latin, um, and, uh, uh, a machine learning algorithm can go through and find the privileged documents without doing an exhaustive search, uh, doing the keyword searches. And one of the examples that I, I think that, that we, uh, talked about, uh, was, uh, a client of ours had, uh, done a privileged review on a set of documents, and they looked at 2.4 million documents and, uh, the company came to us and said, is there a better way to do this? And we showed them how to do this, uh, machine learning iterative approach, and the next review, they only had to look at 40,000 documents. So the cost savings, the time savings, uh, were dramatic.
Speaker 2:That's tremendous. That's tremendous. And, and, and it's an important tool in, uh, a lot of, uh, the, the legal and compliance work that we're currently doing. How are you applying these tools and techniques to healthcare compliance in particular?
Speaker 3:I, I, I think the, the best way to look at that is through, uh, and I'm sure Jerry will have something to say on this, but I is through, we, we think there's been sort of three phases of, of being able to do effective compliance programs. The, the, the first phase was mainly, uh, whistleblowers and people saying that there's been, uh, a problem here. And then lawyers would go look at it. The second phase was searches where you're searching lots of documents and lots of information trying to find problematic transactions, uh, which, which was a, a huge improvement of the first phase. But, but the problem with searching is you can only search what you know for know exists. Um, and so the third phase now is using advanced predictive analytics to look for things that are anomalous that maybe haven't been identified before. If there's a new scheme that hasn't been seen before, that's where predictive analytics can, can find things as well as doing things, uh, uh, less expensively and, and faster.
Speaker 4:Yeah. And, and maybe to, to add to that just a little bit that from a burden standpoint too, if, if you're trying to execute an auditing and auditing and monitoring program and looking at transactions, looking at documents and using search, the ability to differentiate what's coming back from your search I is limited. You come up with 10,000 hits on your search terms, and now you're gonna sample those 10,000 documents you're gonna plow through and look at all 10,000 of those documents. One of the things you can do with machine learning really effectively is a, a more structured form of risk ranking. What's coming back from the, from the program. So you can actually say, let's look at these higher target areas first, let's let, let's prioritize these documents over the less valuable documents on the list.
Speaker 3:One of the fundamental approaches in, in this is, is to rank from most anomalous to least anomalous. And, and the theory being that you can get to the most important stuff early, and that eventually at some point, you, you, you, you run out of problematic transactions. And that's how the, the, uh, the corporation was able to look at 40,000 documents instead of 2.3 million because they ran out of, uh, high risk documents or high risk transactions to look at.
Speaker 2:What I'm hearing is that this represents the next evolution then in compliance program effectiveness. We talked about, uh, you know, the third generation, the whistleblower, um, response being the first, uh, uh, uh, sort of innovation or phase the second being that proactive search. And now it's a pre, it sounds like it's preventative technology or preventative work, um, that, uh, data analytics can allow. Would you, would that, would you agree with that?
Speaker 3:I, I, I, I think that's exactly right. The, the, the, the, the techniques and machine learning that are available right now are, are, are dramatic change from, from the prior techniques. And, uh, I think Matt, you and I had talked about earlier that, that I had seen a, uh, um, seen a, uh, interview with a judge. He said that he thought that, uh, um, keyword searching on its own, uh, is is close to legal malpractice because people understand it. So they do it, but it's so less effective than using, using more advanced techniques. I, I, you know, I, I, I think the world's changing and, and people are figuring this out.
Speaker 2:Absolutely. That's, that's interesting insight. And, um, you know, the reliance on keyword searches is just such a common practice, um, for lawyers and for others in the industry. Um, how are you incorporating this into your representations, your BRGs representations, uh, uh, of medical technology clients?
Speaker 4:Again, in the, in the world of auditing and monitoring, in the world of looking for, um, fraud and abuse, it, it really is a matter of building the, the machine learning algorithms to identify those rather than relying purely on search. And that one of the advantages there when you think of your compliance program and the types of approaches you're using, is, could it help you, um, substantiate that you, the effectiveness of your program, could it help you substantiate that you're looking for the evolving nature of transactions within your business, as opposed to, as Craig mentioned earlier, with search, you're looking for the known activity, you're, you're looking for the patterns that you've already identified that they exist. Um, and that what ML really allows you to do is to look in a, uh, less blinded way. It allows, it opens up, um, the view to new patterns, new types of transactions, new types of interactions that you might not be looking for, and you're gonna lay eyes on those sooner and try to understand them, which again, as Craig said before, it doesn't necessarily mean they're all bad. Maybe through using your subject matter experts and the collective intelligence, you'll end up saying, well, we now understand these because of the evolution of the business, but you've identified them and now you get them incorporated into the modeling. Um, whereas previously you might not have even known they exist. And if they are something that is potentially problematic, you're, you're gonna be looking at it sooner.
Speaker 2:And how, how, how are your clients reacting to not only, you know, the findings, which I think is a, that's such a huge issue, you know, the, the, the, the more detailed, more nuanced findings that you're able to deliver. But how are they reacting also to just the, you know, the incorporation of this type of technology into, you know, perhaps a, you know, a, a tra more traditional audit engagement or a more traditional, you know, compliance program review engagement? What's the reaction and, and, and how do you respond to those reactions?
Speaker 3:You know, I, I, I find the reaction from corporate legal departments to be very accepting. Um, and I, I think there's many reasons for that. I, I, I, I, I think the benefits of this approach, uh, accrue to the corporation, you know, less money, uh, faster times, uh, and frankly, most sophisticated companies out there are using ML somewhere in their organization already. Um, and so it, it, it's not quite as scary to them. Uh, but the other thing we do from, from an approach is not ask anyone to just blindly trust that this is gonna work and that this is better. So we do a lot of proofs of concept, uh, where we'll go in and, and take a limited problem, uh, and, and demonstrate how the technology works and, and what does and doesn't. Uh, and I think that, uh, the usual response from our clients are that, uh, sort of incredulous that, that, that we can get the results that we get.
Speaker 2:That's interesting. And, and, and, and what sort of data would you show them to, you know, to kind of get them past that incre?
Speaker 3:Well, I think if, if, if, if you look at compliance transactions and, and you could say, uh, doing it the normal way in search here, here are, as Jerry said, here, here are 10,000 transactions we're gonna go through and, uh, um, see what we find as opposed to a risk ranked, uh, list of the transactions. So he, here, here are 50 transactions, and they're in order from most problematic to least problematic. Uh, now imagine if we did this on, at, at scale, uh, the, the amount of transactions that could be looked at that could be dealt with, that could be identified very early in our process. Um, um, I, I, I, I, I, I think that's, that's sort of the ticket and it's not really hard to demonstrate. You can put osis information into, um, into spreadsheets. We, we have an analytics dashboard we use so that people can look at it and, uh, uh, un understand it basically in real, real time. Um, we've got an anomaly detection, uh, uh, program that, uh, allows you, allows an end user to try out different algorithms, um, to see what kind of results come back under each algorithm.
Speaker 2:So you've talked about the ability of AI and machine learning to, uh, predict, to prevent, to respond. Uh, you've talked about cost savings, you've talked about speed and efficiency. You've talked about the substantial substantiating, the effectiveness of a compliance program, uh, the evolution of tools, uh, uh, uh, that the compliance officer can use. But my question as a compliance officer, is that the growth of artificial intelligence and machine learning in this space, um, well, I have a job in a few years.
Speaker 3:<laugh>, well, I can't guarantee you anything, Matt, um,<laugh>, But, uh, our viewpoint is that, um, jobs are gonna change. Uh, i, I did a litigation a few years ago where we had 800 contract lawyers looking at documents all at the same time. Uh, that type of rote uh, high volume stuff is gonna change, but, uh, but I think it's gonna open up more opportunities to do more interesting work. I think the, the example that I use a lot is in the early eighties, uh, spreadsheets were just coming into, uh, into the public domain. And, uh, unfortunately, I'm old enough to remember this. There, there was quite a panic on what would this do to the accounting industry or all the accountants gonna be outta work. Um, and the fact of the matter is, today, there's three times as many accountants as there were in the early eighties, and it's because we can do so much. Wow. You know, nobody was thinking about forensic accounting. Nobody was thinking about different types of data modeling that are, that are done now. They were just trying to get the payroll out. Um, and so it, it, it, it, it changed jobs. Um, but it, it, it opened up more jobs than it eliminated. And I think the same thing is gonna happen here.
Speaker 2:Well, that's, that's, uh, that's certainly, um, uh, uh, you know, a, a satisfying response from my, my angle. And, uh, you know, as a compliance officer in the medical technology industry, obviously we deal with artificial intelligence, machine learning, et cetera, in terms of the technology that we produce. Uh, and so this conversation today about how to use those similar, uh, technological tools to actually do our jobs and to do them better, uh, is, uh, really fascinating and, and, and exciting, uh, for the profession. So really appreciate that, Craig. Jerry, any other final thoughts for our listeners today?
Speaker 3:I think one thing that, that, that we feel very strongly about here at B R G is that, um, that products are the wrong way to go in terms of machine learning. We, we, we think that you get a better resolve by having data scientists looking at the problem with the client's data and, and building a solution that way, rather than buying a product and trying to slip it in. And I, I, if you think about it, when a company makes a product, um, the, they, let's say they have 250 use cases, they try to find an algorithm and approach that's gonna match as well as they can to all two 50. And so what that, what that means is you are coming up with sort of a median solution by definition. And if you want an optimal solution, you, you, you've gotta have people thinking about how do I combine different algorithms together? How do I, you know, there's hundreds of settings for every algorithm. How do I test all these things and how do I get an optimum solution? And we feel pretty strongly that, uh, I know this is a little pithy, but, but that you can't separate, uh, data science from the data scientists. You need to have both.
Speaker 2:Well, thank you very much. Craig Freeman and Jerry Lewandowski of B R G. We really appreciate your time today and your insight. Uh, and for our h l a listeners, please stay tuned for more information on further opportunities, uh, in the near term to discuss artificial intelligence and machine learning with Craig and Jerry. Much more to come here. Thanks so much everyone, and have a great day. Thanks, man.
Speaker 5:Hey, thanks Matt.