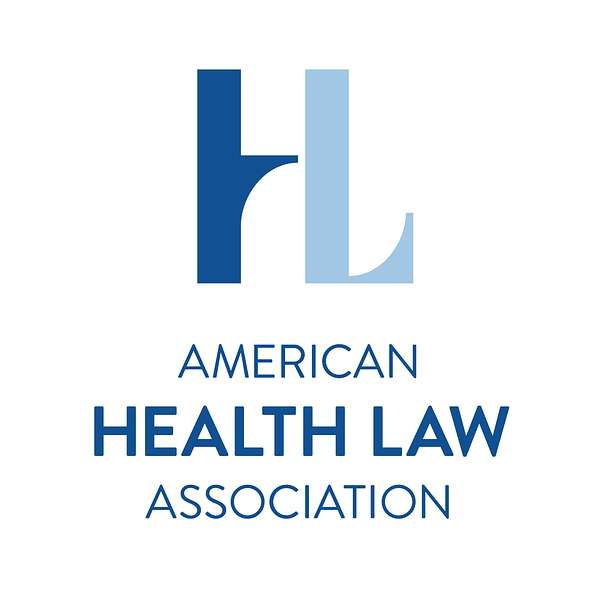
AHLA's Speaking of Health Law
The American Health Law Association (AHLA) is the largest nonprofit, nonpartisan educational organization devoted to legal issues in the health care field with nearly 14,000 members. As part of its educational mission, AHLA's Speaking of Health Law podcasts offer thoughtful analysis and insightful commentary on the legal and policy issues affecting the health care system. AHLA is committed to ensuring equitable access to our educational content. We are continually improving the user experience for everyone and applying the relevant accessibility standards. If you experience accessibility issues, please contact accessibility@americanhealthlaw.org.
AHLA's Speaking of Health Law
Health AI Governance: Navigating the Complexities and Risks
Jon Moore, Head of Consulting Services and Client Success and Chief Risk Officer, Clearwater, speaks with Leah Voigt, Chief Compliance Officer, Corewell Health, and Dr. Mark Sendak, Population Health and Data Science Lead, Duke Institute for Health Innovation, about the policies, procedures, and structures that guide the application of artificial intelligence (AI) in health care. They discuss developing ethical principles and decision-making processes to guide AI use cases, fostering effective collaboration and dialogue about the use of AI, transparency and consent, federal agency and state law developments, ensuring representative data in creating AI, and opportunities and risks. Leah and Mark spoke about this topic at AHLA’s 2024 Complexities of AI in Health Care in Chicago, IL. Sponsored by Clearwater.
New Health Law Daily Podcast Coming in January 2025
Coming in January 2025, AHLA’s popular Health Law Daily email newsletter will also be available as a daily podcast, exclusively for AHLA Premium members. Listen to all the current health law news from the major media outlets on this new podcast! Subscribe Now
Support for A HLA comes from Clearwater. As the healthcare industry's largest pure play provider of cybersecurity and compliance solutions, Clearwater helps organizations across the healthcare ecosystem move to a more secure, compliant and resilient state so they can achieve their mission. The company provides a deep pool of experts across a broad range of cybersecurity, privacy, and compliance domains. Purpose-built software that enables efficient identification and management of cybersecurity and compliance risks. And the tech enabled twenty four seven three hundred and sixty five security operation center with managed threat detection and response capabilities . For more information, visit clearwater security.com.
Speaker 2:Hello and welcome. My name is John Moore, and I'm the Chief Risk Officer and head of Consulting Services and client success at Clearwater, where we help organizations across the healthcare ecosystem move to a more secure, compliant and resilient state. Artificial intelligence is emerging as a potential revolutionary force in healthcare, offering unprecedented opportunities to improve patient experience and outcomes, enhance operational efficiency and drive innovation. However, alongside these opportunities come significant challenges, particularly in ensuring responsible and ethical AI deployment and significant risks and adoption compliance, privacy, and security. As healthcare organizations embrace AI technologies, robust governance frameworks become paramount to navigate the complexities and risks associated with AI implementation effectively. To help advance our thinking about health AI governance, I'm pleased to be joined by two leading voices on the subject, Leah Voight and Dr. Mark Seck . Leah is Chief Compliance Officer for Corwell Health, and Mark is population health and data science lead at Duke Institute for Health Innovation. Leah and Mark spoke about this topic at ALA's conference, the complexities of AI in healthcare back in May. Leah and Mark, it's great to speak with you. Would you like to share a bit about yourselves and some of the work that you and your organizations are doing around ai? Uh , maybe Leo , we start with you.
Speaker 3:Great, thanks John . It's wonderful to be here. Uh , my organization, core Wealth Health, developed an artificial intelligence center of excellence a few years before the pandemic. The COE is designed as a multidisciplinary approach to developing policies and procedures that guide our use of AI and assist in evaluating when, how, and where to implement or embed AI technology in our work. We, of course, pressed pause on our center of excellence during the pandemic, and we reestablished it last year, not long after the release of chat GPT. So now our COE is housed within our data science and analytics department, and participants in our COE work groups include clinicians, researchers, members of our compliance, privacy, and legal teams, as well as representatives from our health plan. Uh, because AI has the ability to transform not only how we deliver care, but also how we pay for it.
Speaker 2:And Mark ,
Speaker 4:And thank you, it's a pleasure to be back with a HLA. Um, there's two hats that I'll describe that I wear. So first it's the role that you mentioned as the population health and data science lead at dhy , which is what we call the Duke Institute for Health Innovation for short. In that role, I work with an incredible interdisciplinary team that serves as an internal r and d group within Duke Health, where over the last 10 plus years, we've looked at opportunities to leverage emerging technologies, care delivery models to solve clinical and operational problems within Duke. So in that capacity, I am a developer implementer product owner for, at this point, it's over 20 different AI applications and products. I will mention that our most recent innovation cycle focused exclusively on generative ai. So we do have a portfolio of about nine projects that are using mostly large language models for clinical and operational use cases. The other hat that I wear is a more recent role. So in 2022, we launched a national collaborative called Health AI Partnership that is a learning collaborative for healthcare delivery organizations to advance the safe, effective and equitable use of ai. And through that role, I work with health systems across the country to surface and disseminate best practices, many of which are related to governance. And then we also are in the midst of launching the first of its kind free technical assistance program for community and rural settings to go through AI product lifecycle management. So have deep experience both at Duke building the products, and then at a national level building capacity to help organizations use these technologies.
Speaker 2:Excellent. So , uh, given the the breadth and depth of both of your , uh, experience, I think there's a lot of things that we could cover today. I I'll try to narrow it down so that we can fit within our, our timeframe. So we wanna talk about , I think we wanna talk about governance today and , and , uh, even governance is probably a pretty broad subject. So let's define or scope down that , uh, that itself for this conversation. So today I'd like to focus on, let's call it governance from the CEO down. So those policies, procedures, organizational structures that would guide the adoption and application of an AI within a healthcare organization, as opposed to what I think of as from the CEO up or the board level governance that an organization might , uh, engage in. Does that make sense for, for you, Leah , mark ? Sure does.
Speaker 4:Sounds great. Alright .
Speaker 2:So with that being said, I'd , I'd love to discuss developing ethical principles and decision making processes to guide AI use cases. So what do you both see as the most important considerations when thinking about , um, AI ethics, trustworthy ai? Uh , there's a number of concepts I think that, or , or different , um, names for , uh, these ideas that are out there right now. Maybe Mark, we start with you.
Speaker 4:Yeah, so , um, maybe I'll give a controversial response initially, which is primarily through health AI partnership. I see a lot of organizations struggling with how to reconcile the different principles that are coming out from the White House, from nist, from different regulatory agencies. You have faves from ONC and I , I'm definitely not gonna take a stance on that. There's one right set of principles. I think at the most basic level, we think about safe, effective, and equitable. So that goes a little bit beyond what the FDA historically has focused on, which is safety and efficacy by incorporating equity. And I think that's really in response to so much work in the last five to 10 years that has shown how algorithms can reinforce and perpetuate biases, disparities, discrimination in healthcare. So I think that we need to go beyond the way that maybe medical devices have historically been tested. And then the work that we do in health AI partnership is to help people understand that whether it's trustworthy, whether it's fair, these principles from different government documents, you can align best practices for governance and product lifecycle management with those principles. And it doesn't require you to do a whole lot beyond what many of the leading organizations are currently doing.
Speaker 2:And Leah , your thoughts in including whether or not you think that's controversial or not? I <laugh>
Speaker 3:Certainly not to me. Uh , but , but Mark and I had opportunity to talk about these , um, topics quite a bit in the last couple months. So Mark , I don't find that controversial. Um, uh, I will add on to Mark's comments, and I'll look at this through that governance lens that, that you described, John, I, I see this as a matter of kind of first things first for healthcare organizations who , um, that are, that are seeking to adopt ai. And I think that to, to simplify that there , the approach to ai, I think there are three main questions or series of questions that I think organizations have to ask of themselves, whether they're in the midst of an AI approach or whether they're, they're still trying to find their way. Uh, I think that first question is what's our organizational strategy and how does AI enable it? I think the tendency for organizations and healthcare in any organization, but I say particularly in healthcare, when there's new technology, there is this tendency to think that we have to have a strategy just for that technology. Um, and, and I think maybe some organizations might have revised their strategy to include ai, but I think for many, if not , uh, the vast majority of healthcare organizations, it's your strategy is really about caring for patients. And so AI is aena an enabler of that strategy. So again, examine your strategy and think about how AI is gonna help you get there. And that's really the second set of questions that I would highlight is, you know, where do you wanna go as an organization? Where do you want that enablement to occur? Um, and what's your risk appetite as you're aiming to get to that point that you define for yourselves? And then the third question , um, and I think Mark really was, was commenting around this, where are you at as an organization currently? Do you have the right foundation , um, to implement AI in a responsible way? Meaning do you have the people, the processes, and the technology? And if you don't, what do you need to build? So again, in other words, what's your readiness as an organization to responsibly adopt ai? Um, I add just maybe two other points onto this. I think that cross-functional engagement , uh, in answering all of those questions that I just outlined , uh, is absolutely key. Along with highest level support from executive leadership and your board, because of the transformational capacity of ai, I think that you can't have this be just a management or from within the heart of the organization , uh, approach. It really has to be top down and from side to side. And then I would say ultimately all of that has to align with your organizational mission and your values. If caring for people is at the heart of what you do and who you are, then that has to drive the decisions you make, including any decisions related to AI implementation. And that key question being, is it going to benefit our patients? I think that the answer to that question has to presume that the AI is safe, free from bias and designed to protect the privacy and security of patients' information.
Speaker 2:I, I think I, I didn't find either this comes particularly controversial, although perhaps some do as well. I, I , you know, I think it's, it's interesting to think of AI as a, as a tool and, and it's a tool just like a lot of other tools that we use in delivering healthcare. You know , it's like a scalpel in some ways, right? It's just another tool. And we can either use that tool effectively in , in accomplishing our mission, or we can use it less skillfully , uh, with a lot of particular consequences associated with it. So I , I really enjoyed , uh, both of your thoughts on that. And stakeholder engagement is another key issue I think, surrounding ai. Mark is a , a physician and a technologist. How can we foster more effective collaboration and dialogue about the use of AI and ensure that we continue to have and maintain that patient trust and how we use their data , uh, how we use these new technologies.
Speaker 4:So , um, I'm gonna start by describing stakeholder engagement within the organization, and then I'll speak more explicitly about patient engagement, which to kind of highlight where I, where I'll go with that is that we're, we're definitely just not doing enough with patients around ai, but within the organization. And, and this really is a, a competitive advantage of the way our team is structured. We're technologists within a delivery system. So to , to Leah's point, my organization has the same mission and values as the same as the clinicians who I work hand in hand with to build and implement technologies. So there is alignment in terms of ensuring quality of care, safety of patients, and the way that we prioritize and select problems that we work on. Where we ultimately, a fraction of the time use AI is we invite clinicians to submit proposals to an innovation competition. So the problems come to us from frontline clinicians who face a problem in their daily practice that they need help with, and things get routed to us if kind of the standard quality improvement process improvement frameworks have not been successful. And there requires kind of a new technical approach to solve the problem. So I see this pretty widely in other contexts where you have a lot of technology built outside of delivery organizations without the buy-in from frontline clinicians. And it creates a lot of friction when you near adoption and implementation. And I would say that the, the earlier you can engage frontline workers to orient the technology around problems that they feel the pain around, the more successful you'll set yourself up for to, to be able to implement and show impact. So that's the engagement of the frontline clinicians, which I view as completely critical and not kind of uniform in the way that it happens. Now, specifically talking about patients, I actually have a lot of concern that we are losing patient trust with how health systems use their data. I am part of a health system that has contracts with third parties where we de-identify and provide data to third parties to build products. Most patients don't know, patients have not been informed. And then in my role as a developer internally, we often build products through QI exemptions, quality improvement. And I would say that I really hope that culture changes. We're trying to influence this somewhat through the work we're doing in health AI partnership, where I would love to see a future where if I receive care in a delivery organization, I have a little bit more visibility into how AI is used in my care, how my data is used to build or distribute AI products. So I , I think that, I don't know what's, if, if the cart's gonna come before the horse where health systems are gonna start moving in this direction before regulation comes out. But I, I definitely see it as something on the horizon that we need to be thinking more about.
Speaker 2:Building on that last question, Leah , and, and Mark's response, I'd like to get your perspective on transparency and consent. Is it practical to obtain consent from the, from the patient, if not consent? What about other efforts at transparency , uh, around where, how their data is being used and, and where it ends up going to? What, what are the options? So
Speaker 3:If you are going to implement AI in ways that ultimately benefit patients and improve patient care, then I think a corollary to that is that patients really need to understand how AI is gonna help achieve those objectives. And the concept of informed consent is rooted in that principle, that to make a decision about one's healthcare, it has to be just that truly informed about the treatment options. And we know that treatment options, of course, depending on the particular patient , um, but , but treatment options more broadly have always involved technology or innovation in some way. And informed consent and transparency in my mind, is just as important with AI as it is with any other new technology or development. Um, especially one with the ability to transform the entire industry. Um, so not everyone is gonna be comfortable with AI chatbots and clinical decision support tools. And , um, on the other hand, some may play , some may place too much faith in the ability of AI to improve , um, healthcare outcomes and experience. So all of that, that kind of as background, I, I would say that in terms of deciding whether to obtain consent, I find it helpful to approach this , uh, in a, in a two part analysis. Um, as, as most lawyers can appreciate , um, we, we have multiple , um, uh, elements or steps in our legal analysis. Um, and that second part I'll talk about , uh, is a little bit of that cart before the horse that I think Mark was mentioning. Um, that first step though in the process , um, is in my mind to ask the question, what is legally permissible and what is practical? Um, and I'll explain here in a moment why I think both the legal and the practical in , in the context of AI really have to go hand in hand . Um, because while legal and regulatory constructs might exist, and they do , um, I think that AI poses some real problems for us from a a practicality perspective. Um, as, as healthcare providers, we have to think through a number of existing legal constructs. Um, your state's informed consent laws, HIPAA authorization requirements , uh, and if the AI is currently under development, you may also have to consider the research consent requirements under the federal common rule and FDA regulations. Uh, and this is where we're gonna run into practicality problems really pretty quickly. Um, because let's say we've identified , um, that the primary reason to obtain consent or authorization is based on the use of patient data to generate a result via artificial intelligence. And under our current legal constructs, the purpose of consent or authorization to use PHI, for instance, is to gain permission , uh, to use the individual's data for a specific purpose or a set of purposes. And if AI by its nature is adaptive, I think a theoretical, and now really practical question is, can an individual truly give informed consent at that first point of contact or that first point of data collection , um, with all of the data that might be fed into a particular AI tool or algorithm as that purpose or use of the tool evolves. Um , what does that mean for that point in time at which the patient first gave their consent or their authorization? I think another practicality problem is even if you need to or you want to obtain informed consent, how do you operationalize that? Meaning how do you have the conversation with the patient or their representative? How do you document that? And then how are you able to track it? Um, if we're talking about things like clinical decision support tools that are actually embedded in our medical records, embedded in the care process, getting consent at every point in time that that tool may be at play is probably not practical. Um, by comparison, if we think about AI chatbots that are enabling communication with providers and enabling access to care, you know, maybe that's, maybe consent is more practical , um, but maybe it's not practical each and every time that an individual might interact with that tool. So I think that legality and practicality actually go hand in hand . Um, and then the second part of this analysis, and I think Mark was really getting at this, is that even if consent might not be required, there is still that very important question. Is it the right thing to do? And if it's not consent or something that looks like consent, what are the other options for transparency? And I think there are a couple of different things that organizations really should be considering. Um, the first being right at that point of care. This is where our provider education and understanding is just so important. And I would add to that provider willingness to really engage in dialogue with their patients , um, about AI and the way in which AI is being used today , um, to help , um, create efficiency , um, and accuracy in the care that we provide. So it's that patient provider relationship that I think is absolutely crucial. I think another consideration is really at that organizational level , um, talking about it , uh, whether that's through patient facing communications on websites , um, notices or articles in patient portals or in social media articles that might be sponsored by the organization. I also think there's an opportunity if the organization chooses to do so, to really talk about their AI approach, their AI journey publicly in, in healthcare focused and , and other media outlets. And I think that all of those transparency considerations that I just outlined are , are not mutually exclusive. In fact, if anything, I think that they should be layered on. Um, and it should be , um, a yes and approach.
Speaker 2:So you flung open the, the legal or regulatory door. Leo , let's take that , uh, a little bit further. So , uh, in my impression anyway , is that regulatory requirements related to the use of AI in healthcare are, let's say, evolving. Uh, you know, as we, as we talk here today , um, we have ONC, which I, I guess is either now or soon to be renamed as the Assistant Secretary for Technology policy in the office of the National Coordinator for Health Information Technology. Um, we have asper , we have FDAI , I think that Mark mentioned earlier, we have OCR from a , from a HIPAA compliance and security perspective as well. Um , how are these different , uh, organizations within the healthcare regulatory ecosystem , uh, you know, how are they addressing AI regulation? And then also to the extent that you can comment on it, you know, how are state laws as well impacting the use of ai ?
Speaker 3:Sure. So , uh, you mentioned OMC right out of the gate. Um, OC is regulating AI developers, particularly those that create clinical decision making tools that are embedded in our medical records. Um , O C's recent ht one final rule, which implements part of the 21st Century Cures Act actually includes transparency requirements for artificial intelligence , uh, and other predictive algorithms that are part of certified health. It , uh, and as you noted, John, I think now that ONC has been reorganized to include this HHS wide role of the Chief AI officer, I think it's gonna be very interesting to see how this agency continues to lead on AI regulation. Um, we've also talked here today about the FDA. Um, the software as a medical device guidance has been out for many years now, and a good number of AI products have already been approved or cleared under that guidance. FDA is also considering regulation focused on the quality and the track record of the developer versus the safety and effectiveness of the particular technology. Um, one of the challenges with the current state of FDA regulation of medical devices is that it is static, meaning that review and approval by the agency is based on data supporting a specific use of the product, kind of a point in time, if you will. Um, but AI products, as we know, by their nature, are dynamic and evolving, so applying this type of framework really isn't feasible. Um, so keep watching FDA, I would say , uh, as well. And then the other agency, I would know , the , the Office for Civil Rights. This is more of a question in my mind about , um, AI and how the regulations that are enforced by OCR, how those things interplay. And it's not because HIPAA doesn't apply to ai , um, but because OCR has seen to signal that it's not considering changes to the rules or updated guidances to address this technology at , at least not to this point. Um, but I think, I think time will tell on, on that agency , um, as well. Uh , I'll speak , um, briefly on state laws. Um, as you noted those as well, John, to date, there are really very few state laws specifically addressing or regulating ai. I think states generally understand that legislating in this area will ultimately lead to a patchwork of requirements that, although they may be well intended , um, will be really unworkable for AI technology developers as well as consumers. The one area of state law that I will note , um, that is with respect to laws pertaining to consent to recording conversations, many states have laws that require consent by one or more parties to the conversation before it can be recorded. So for instance, in my state of Michigan, we have a one party consent law. So , um, only one party to the conversation that needs to consent to the recording. I raised this issue because one of the AI technologies that's really exciting for healthcare is ambient listening and conversation platforms. Uh, they can record patient provider interactions and then generate the, the corresponding medical record documentation.
Speaker 2:Uh , thank you. I, you know , in thinking about OCR and, and , uh, hipaa, there's of course also the more of the civil rights focused area. And , and Mark, you, I think, mentioned equity early on in our conversation today. You know , I think about data quality , uh, and , and as another big consideration, mark, what recommendations do you have for ensuring, let's call it representative data in creating AI and assessing the quality of data after it's generated?
Speaker 4:So I've spent a lot of time cleaning data . <laugh> <laugh> , the first five years of, of working at DHI was really just creating reproducible processes and a bit of technology so that we could curate high quality data more rapidly to either build novel or test existing AI machine learning products. So I just can't emphasize enough how important data quality is to any effort to prepare for implementation and during implementation, because healthcare data is just so dynamic in terms of the way tests are ordered, medications that are prescribed patient populations. I , I know we, we mentioned putting things on hold during COVID . Um, during covid , our hospital wards were reassigned different levels of care. So one week ward would be a, a step down unit the next week, it could be an ICU, the next week it could be a, a perioperative unit. So anything that we were doing with , um, transitions in care and escalation of care, we had to be extremely closely monitoring our health system operations. So data quality just extremely important. The question about representative data, I'll give an odd response, which is the most important thing in the implementation context is how well does an AI product work within that context? So there are higher resource sites like Duke being one of them, that we build many products on our own patient populations. So by the nature of how the product is built, it will be representative of the types of patients that we care for. That is unusual. Most healthcare delivery organizations will use products that are built externally by vendors. And in those contexts, it's gonna be very important for, I would encourage every organization to look at doing some amount of local validation of the product with patient cohorts that are representative of their own context. So I, I'm not answering the question directly by saying, here's a way to curate representative data so that you can provide performance assurances in every context, because I don't know if that's possible. I don't know if it's feasible for one developer to get such representative data that you don't then need to do local validation. So I, I more wanna point folks towards curate a representative data set of your own population to evaluate any externally built model so that you can feel assured that the product performs on your population.
Speaker 2:So we're about out of time, but I wanted to, to give you both an opportunity to, to address , uh, one more question. That's actually probably a compound question, but what do you both see as the most compelling opportunities right now for health ai? And conversely to that, what are the key risks that need to be managed , uh, as, as part of our further adoption of ai? Leah , do you wanna start?
Speaker 3:Sure. Um, so you sitting here today with both of you, I think the most compelling opportunity is to reduce the administrative burden on our providers, actually , uh, tools that help generate notes. Um, patient consult summaries help create email responses to patients. Those are real game changers , um, helping to reduce pajama time and improving the wellbeing of , uh, those who care for the rest of us. In terms of risk , uh, we've talked about several of those key risks already. Safety and accuracy, bias, privacy, and security. I also worry about the eventual loss of critical thinking and human connection. Sometimes the data can't tell you everything about the patient sitting in front of you. You have to see them interact with them and, and use those human skills that simply can't be replicated by technology. Um, I I would also comment a , a bit on third party risk, and John, we could have another podcast dedicated entirely to this topic for sure. Um, what, what I would offer on this point is that organizations should really make sure that their work around managing the risk of ai, especially if, as Mark noted, it's going to be sourced externally, that that work is embedded with, or part of what the organization already does to manage third party risk more broadly. I think there's an organizational tendency to develop AI governance and oversight on an island or as a special initiative, but I think we can all agree that AI is here to stay. So, going back to the start of our conversation, when I described our AI center of excellence, I think the key is to make sure that the policies, the processes that are developed by a group or a team like A COE , really in sync with vendor risk assessments and risk management activities that exist.
Speaker 2:Mark your thoughts.
Speaker 4:So I completely agree around the opportunities for reducing administrative burden. I think I also have the, the viewpoint internally where we've worked on so many projects to support clinical decision making in chronic disease management, in acute care, deterioration detection and management. And I know that , um, there's a lot of folks who are wary of those more high risk patient bedside use cases, but the reality is hospitals still have trouble detecting and treating sepsis. Hospitals still have trouble responding to acute care deterioration, and there's such a huge opportunity to leverage data, so wanna make sure that those opportunities are kind of pursued. The , the other, the risk that I see that we're working pretty proactively on with health AI partnership is the digital divide. And I think Lay has alluded to this. Duke can have a center of excellence. Corwell can have a center of excellence. There's many, many federally qualified health centers, I mean, 1600. There's community hospitals that may not have the interdisciplinary expertise to stand up their own internal centers of excellence. So really trying to think about how do we diffuse expertise outside of these centers of excellence so that you can see a hub and spoke model that we see in other areas of healthcare, where highly specialized centers diffuse expertise and extend expertise to rural and community settings to provide support around complex technical or medical conditions. So thinking proactively about what types of policies and funding structures do we need to provide that support to bridge the digital divide that we have currently.
Speaker 2:Thank you, Leah and Mark, for your excellent insights you shared. Uh, today. I, I hope we can talk more about number of the issues, you know , number of the things we've discussed today, sometime again soon. Uh, and thanks to our audience for listening today. I hope you have a great rest of your day.
Speaker 1:Thank you for listening. If you enjoy this episode, be sure to subscribe to a HLA speaking of health law wherever you get your podcasts. To learn more about a HLA and the educational resources available to the health law community, visit American health law.org .