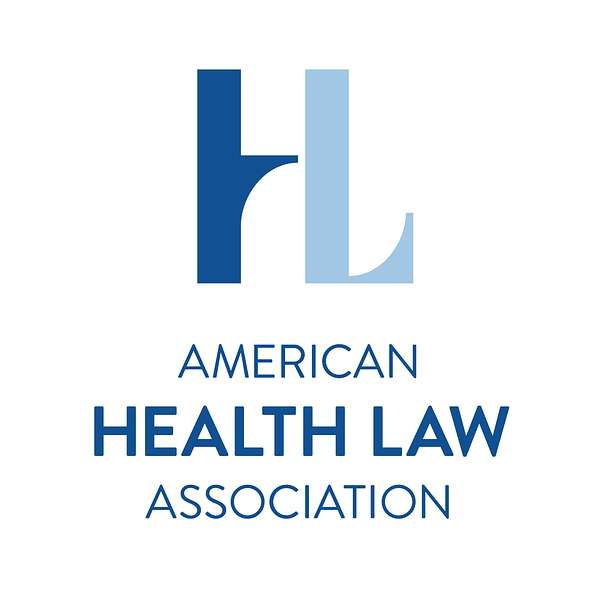
AHLA's Speaking of Health Law
AHLA's Speaking of Health Law
AI in Health Care: Case Law and Enforcement Trends
Nathaniel Mendell, Partner, Morrison Foerster, Kristopher Hult, Principal, Charles River Associates, and Jonathan Porter, Partner, Husch Blackwell, discuss some hot topics related to artificial intelligence in health care and accompanying case law trends. They cover issues related to antitrust, patient facing applications, claims processing and claims maximization, and enforcement efforts. Nathaniel, Kristopher, and Jonathan spoke about this topic at AHLA’s 2024 Complexities of AI in Health Care in Chicago, IL.
To learn more about AHLA and the educational resources available to the health law community, visit americanhealthlaw.org.
<silence>
Speaker 2:This episode of A HLA speaking of health law is brought to you by A HLA members and donors like you. For more information, visit american health law.org.
Speaker 3:Hello everybody, and welcome to this edition of the A HLA podcast. Today I'm joined by Jonathan Porter and Chris Holt. We are going to , uh, present to you about a panel we gave at , uh, the A HLA conference in Chicago back in May. Uh, the topic was complexities of AI in healthcare, and we talked about some patient facing , uh, aspects of healthcare as well as antitrust implications , uh, of AI in healthcare. The panel was great, I have to say , uh, mostly because the audience was very engaged. There were many questions, and, and I think that was just because we have , uh, such a good topic here, very timely, and , uh, plenty of things to, to discuss. Uh, before we get into the details of what we covered on the panel. Um , Chris, Jonathan , why don't you guys introduce yourselves?
Speaker 4:Sure. So, I am Chris Holt. I am , uh, an economist , uh, in the antitrust space. I work at Charles River Associates in Chicago, and I am a principal there.
Speaker 5:And I'm Jonathan Porter . I'm a partner with Hush Blackwell. I'm , uh, I'm a former federal prosecutor, and now I'm in hush blackwell's healthcare and white collar groups, and I'm really excited to get this band back together.
Speaker 3:Thank you, Jonathan. Yes. I should probably introduce myself too . I'm Nate Mendel. I'm a litigation partner at Morrison Forrester . I work in our Boston office. Uh, and before returning to private practice, a couple of years ago, I was the acting US Attorney for the District of Massachusetts, where I was a federal prosecutor for almost 15 years. Um, so I have to be at Morrison and Forrester and , uh, like you guys, I think, happy to get this band back together. The panel was good and , and , and the event in Chicago was a good one. Alright , uh, why don't we start with you, Chris, you, you talked about antitrust and, and explain some of the ways in which AI can really create some, some difficult issues , uh, in the healthcare space. So , um, why don't you take it from there and, and start us off?
Speaker 4:Sure. So one of the things , uh, there , there's I think a lot of ways that we think that AI can affect healthcare. Um, and one of the ways that AI can be implemented in healthcare is by setting prices. And one of the things that AI can do is that it can take a lot of information and process that , uh, very quickly. And so one of the ways that it can , uh, apply that is taking lots of data, both sort of historical , uh, and real term , real-time data, and use that to predict demand for consumers and health firms to set prices , um, not only in healthcare, but in all sorts of industries. And , uh, one of the big concerns that arises , uh, from this use of AI is that these pricing algorithms , uh, can lead to collusive outcomes where firms are collaborating in some way to gain an unfair market advantage. Generally, we think about , um, these firms raising price by manipulating market outcomes.
Speaker 5:So, Chris, I'm curious, how exactly would ai , uh, play a role in this risk for collusion?
Speaker 4:So there's, I think, two main ways. One is what we call ta collusion. That's collusion. Where when we think about collusion, a lot of times you think about sort of people getting together in a smoky back room and shaking hands and agreeing to raise price. Um, but collusion can occur without sort of a formal agreement. And when you have collusion where you're not sort of directly agreeing to include , but you create sort of a scheme in which , um, prices are manipulated, that's called TA occlusion. Um, so that's sort of one area. And the other is from third party pricing algorithms. 'cause a lot of this ai it's not the firms themselves who are sort of doing the AI themselves and running the , uh, analysis. They're gonna be outsourcing to, to third parties who are creating and implementing these pricing algorithms using ai.
Speaker 5:So Chris, I'm super fascinated about this. I don't know about Nate, but I'm, I'm very interested in how AI could get into these smoky rooms. So why don't you tell ALA's listeners about how AI could learn to collude. How does it get into this tacit collusion area?
Speaker 4:Yeah, so the tacit collusion has been one of the more interesting areas. 'cause there's been , uh, some research that's shown that if you train these models , so ai, you give it a lot of data and it's sort of is a learning model where it's training how to set prices. Um, these, these AI models can actually sort of learn the reward and punishment schemes of collusion by themselves, sort of without even being explicitly told. Uh, and that's a potential concern. And so we see that, you know, if you tell a , uh, an AI model to , to set price in the way that sort of maximize prices for the firm , uh, it can learn behaviors that we would often can think would be , uh, antitrust violations or , um, behaviors that are raising prices above what we would expect in a competitive market.
Speaker 5:And then one , Chris, one of the things that you talked about when we were in Chicago together was how third parties play some sort of a role in, in , um, in, in creating antitrust concerns. So , so tell our listeners here a little bit about what you told our, our audience in Chicago when it comes to third parties running pricing algorithms and that creating , uh, antitrust concerns.
Speaker 4:Yeah, so one of the big issues always with collusion is coordination. So you have to , uh, keep the firms working together. 'cause there's always an incentive if someone gets together and say, Hey, let's raise price. There's always incentive for one firm to deviate from that , um, and try to undercut the firm and , and take consumers away. And so having a third party setting prices , uh, really helps that coordination problem. It it , one, it facilitates information exchange between potential competitors, but it can also be the case that you have one firm setting prices for multiple competitors. So if you think about a drug or , um, you know, different services in the, in the healthcare space, and there's sort of major players who are doing this price setting and there's lots of drug companies , um, or hospitals getting together with a single or even a small group of , uh, firms that are setting these third party firms that are setting these prices, that creates a much greater incentive to include . And also makes it a lot easier for firms to collude because again, you have someone that's sort of dealing with both, both parts , uh, of the market, both competitors or , um, you know, has information across a variety of firms, which you wouldn't generally have in a market.
Speaker 3:And Chris, one thing that we talked about on the panel we , we've all seen in our work is that ai, it , it, it adds something to the equation, sort of turbocharges it , um, and makes things go more quickly or more comprehensively. How does that factor into the, the price setting dynamic? Like what is it about AI or how it's used that that makes it different than just everybody using , uh, the same consultant?
Speaker 4:Yeah, the , I think the , the biggest thing is that it's really , uh, it's very efficient and can do a lot that when we think about sort of individuals at a firm setting price, it can take a lot of information and process that very quickly. And so , uh, it has the ability to think about sort of lots of things at one time. It can react quickly , um, to changing market conditions. It can deal with sort of lots of data , uh, in a way that , uh, uh, people running the running pricing by their own couldn't do it quite as quickly. And, and the other thing is, it's also just a lot harder to , um, determine whether collusion's happening and what the algorithm's doing. And so if you have, you know, specific pricing groups within a firm , you can sort of see if there's interactions. You can sort of see how they're thinking about pricing. You can, you know, there's some paper trail of how was this price set, you know , how do they incorporate maybe their competitor's information? Uh, and with ai, we don't really have that the same way. And so it can be a lot harder to tell is this , uh, algorithm, what kind of data is it using? Is it considering the other firm's prices? It's much more of a black box. And so I think that poses an increased challenge , um, both the firms who aren't sure what to do with it, but also to regulators trying to figure out how do we sort of prevent these , uh, potential antitrust issues. And I think that's one of the, the big concerns is just how do we, how do we handle that?
Speaker 3:Yeah. The former prosecutor in me is imagining a lot of , uh, frustrated investigators and prosecutors getting the answer that, you know, the algorithm said I should do X and that's what I did. Yeah. Where's , where's the intent? Where's the C enter ? Right.
Speaker 4:And there , and there can be no communication too, you know, a lot of collusion cases, there's some emails or some conference where you can say, this is when they got together, and we can sort of look after that. Um, but it's, it's just very hard 'cause you don't have sort of a specific program. You don't have a lot of paper trail. It's hard to say, well, how did you set, tell the ai , how did you set the price, you know, two years ago on for this service? Uh, you know, give us a specific sort of breakdown that a a judge or lawyers can understand.
Speaker 5:Yeah, I'm the , I'm the same as Nate, my federal prosecutor mind works very simply in terms of what can I explain to a jury? I don't know how you're gonna explain to a jury that AI was used to drive antitrust violations. That that seems really hard, Nate , uh, uh, but actually, Chris, I'm curious. Have, have you , you , 'cause you're, you're in the weeds on antitrust. Have there actually been cases like this already where you've seen AI driving , um, driving antitrust concerns?
Speaker 4:There have been cases that are sort of about pricing concerns? There's been a little bit, but it's really, this is sort of the start of ai. You know, there's not , um, well developed case law yet in this area. And I think we'll just see more and more cases. Um, there has been a case , uh, in healthcare and MultiPlan, which is , uh, a re a , a company that sets reimbursement rates for a bunch of , uh, insurance companies. And so that's a situation where you have lots of insurance companies relying on sort of a single party to set prices. Um, and so they have been sued for antitrust , uh, violations. And I think people are watching these cases and what kind of cases are gonna come out? 'cause it's more, with a lot of this AI stuff, it's more what's going to come than sort of what's already on the docket.
Speaker 5:Yeah. I, I , I think this is a super interesting area, and one of the areas that I've heard is, is where health , um, is where health plans are sort of, they have more operations than just their health plans. Um, and where AI is, is, is being used to drive denials. Um, and those denials may help one of the , um, one of the payers , um, various, you know, medical lines. I I think that's a really interesting antitrust concern. And, and so, yeah , uh, Chris, I think this area is, I think you're gonna be very busy , uh, in , in , in the future in your practice, because this is gonna be continuing to be a , a huge, a huge thing. So , um, changing topics a little bit, Nate, we, when we were in Chicago, you, you talked a lot about , uh, patient facing applications. I think there's, AI has tremendous promise when it comes to patient facing applications. There are a lot of instances in healthcare where , um, if a patient can interact with like a chat bot or something like that, it can, it can create great results. And you had an interesting example of, of that. So, Nate, do you wanna tell our, our listeners a little bit about , um, that
Speaker 3:Yeah. Thank you, Jonathan . So, so the , um, I think the audience pleaser example that , uh, that I used was , um, a pilot program at Brigham and Women's Hospital in Boston where ai , uh, is run during patient visits with the patient's consent, of course, which apparently , uh, is given at a very high rate. And what the AI does during the visit is basically , um, kind of drafts up , uh, a summary of the patient visit for, for the doctor. Uh, it's done, man . If it's done manually, it's very labor intensive. It can be kind of distracting. I think we've all had an experience where we're in the doctor's office and the doctor appears to just be typing into a PC and periodically asking us questions. And it's, it's, it's, it's a little awkward. And apparently for physicians also, it's just , just kind of very much in the way. So AI solves that problem and generates , uh, these summaries, which , uh, according to the pilot summaries are actually quite accurate. Uh, and it relieves physicians of a , a massive administrative burden and allows what we all would want, which is a very direct , uh, doctor patient connection. Um, so, so the Brigham was, I think , uh, a success story where , um, a relatively simple idea is made possible by ai. Um, but you know, Jonathan, you know, as well as I do, right, that those kinds of innovations and powerful tools, right, in a doctor's hands , um, they can be really good, but whether they're good or bad, they're, they're just really effective. And I think you talked about a case which maybe as a template for future AI investigations and prosecutions where the power of algorithms and AI was used in maybe a less positive way .
Speaker 5:Yeah, absolutely. Nate. Yeah, so I think what's interesting, there's two big things with AI and healthcare that are, that, that I think everyone should be thinking about and saying, these would be amazing for the healthcare industry for patients. One is, one is, is patient facing applications, you know, the , the the chat bot thing, which I think is, is super interesting. And by the way, there was a study done not long ago that showed that , um, AI actually has better bedside manner than the average md, which I think is fascinating. Um, but the other thing is clinical , uh, decision support. So clinical decision support is something that a lot of health systems are already using. It is a way for , um, it is a way for some pe for some physicians to receive little pings when there are , uh, things that could be done. And so it , it's, it's largely driven by algorithm. But I think if you were to sort of turn that over to some sort of an AI model, the pro the , the promises would be even greater. The theory in , um, with AI in healthcare, and you, you hear, you know, NVIDIA's, CEO talk about this earlier this year at a conference , um, is, is that if you give AI a bunch of data, it's gonna find better ways of practicing medicine. Um, and clinical decision support is like the model that I think AI could really grab onto and, and, and make it great where you are letting ai , um, not just do its diagnosis thing, but also suggest courses of treatment courses of, you know, additional tests that you can run to figure things out. The concern there being this practice fusion settlement from a few years back, which is really, really interesting. And, you know, definitely worth flagging for our listeners here. So Practice Fusion was a clinical decision support tool. Um , but what it was doing, it would ping doctors when , uh, certain events , um, were , were , were coming up. So in, in the, the specific example here is it would, it would alert doctors when it thought that perhaps patients needed more pain management. Um, where Practice Fusion got into trouble was a particular opioid company was sponsoring its program. It was sponsoring his program. They Practice Fusion allowed this opioid company to be involved in how the alerts were set up when the alerts would go out. And so Practice Fusion was , um, was investigated and ultimately pled guilty and , uh, agreed to an FCA settlement. The FCA settlement was I think, $118 million. Um, as part of the criminal plea, it agreed to $26 million in fines and forfeiture. Um, and so the, the concern here is if you're going to design this AI model for clinical decision support, you cannot take , um, kickbacks, you can't have sponsors and let those sponsors drive the events where you're gonna hear this clinical decision , um, alarm go, go off. And so I think this , um, I think that Practice Fusion case is, like you said, Nate, an excellent template for what could go wrong in the future when AI is involved and there are others. What do you think about that, Nate?
Speaker 3:Yeah , the , the Practice Fusion case , uh, like a source of endless fascination for me, maybe because I, I have a simple mind, I'm not sure, but I think it might be because you see there really this , um, such a powerful example, right? Where if you had AI running, it would never forget anything in a patient's file. It would, it would never forget , um, like a , um, drug interaction issue. It , it would, it would catch all of those things, which has to be better for patient safety. Um, but then you see, you know, the risk of the human element where , um, if you, if you tweak that algorithm to generate more revenue for a particular supplier , uh, obviously that's, that's an a KS issue. That's a false claims act issue. But, but how are our former colleagues ever gonna find that? It's always gonna take a whistleblower? So it, it's a, it's a healthcare investigator or healthcare prosecutors' kind of worst nightmare that, that these issues would be occurring. And it , it's, it's just totally invisible except to the real insider.
Speaker 4:Yeah. And I think that gets sort of, that a lot of this AI stuff is sort of outsource, you know , get these third parties who are introducing more problems. 'cause it's some other firm that's coming in and making these decisions. And , um, without transparency, that can cause a lot of issues in healthcare.
Speaker 5:Yeah. A a , absolutely. So let , let's, let's change topics a little bit again, Nate, why don't you tell our listeners a little bit about claims process and claims maximization. I think this is a really interesting area when it comes to AI and healthcare claims maximization, and there's a case that I think , uh, our, our listeners would love to hear about too.
Speaker 3:Yeah, definitely. And , and it is, I , I mean , um, the examples that we're talking about all have a certain similarity, right? Where if you could optimize a system that would be great . Uh, but the same tool that you can optimize a system , um, you know, sort of within the rules could also be tweaked a little bit and go outside the rules and optimize in a way that is recognized as impermissible under all the kind of familiar , um, rules we have in, in governing healthcare. Uh , so the , the particular case , uh, Jonathan , to answer your question , uh, senek , the Kaiser , uh, and it's in the northern district of California , um, it's a whistleblower case. Uh , uh, the government has intervened. And what I'll talk about is the , the allegations that the whistleblowers are are making. So , uh, there is a , um, well, Kaiser has, of course, many, many patients and, and many, many , uh, uh, patient records. They saw this as potentially a business opportunity looking at that and seeing if they could go back and find more lucrative diagnoses for Medicare patients to raise that patient's risk score. And those familiar k kind of with the Medicare, Medicare world will know that if you raise the risk score for the patients you're treating, you raise revenue. Uh, and in particular, they , uh, Kaiser has allegedly went back through all these patient records looking for , um, uh, uh, evidence, right? Uh, characteristics of patients that would be consistent with a diagnosis of aortic atherosclerosis, which for civilians you would know as hardening of the artery walls. And if the AI found the indicia of that diagnosis in, in the patient records , uh, Kaiser would go back retroactively to the physicians and pressure them or give them incentives to retroactively change the diagnosis to make it a more serious diagnosis with a higher risk score with more money, more revenue for Kaiser. And, you know, Jonathan, you'll , you'll recognize all of this as it's, it's like back and forth across the line of things that are certainly perfectly permissible to do, like, like coding your activity accurately and capturing all the things that you are really doing. That's very important. But just on the other side of that line, tweaking your coding <laugh> upcoding , uh, inappropriately , uh, and so, you know, organizations get in trouble going back and forth across that line. What I think's really interesting here, right, the allegations are from 2009 to 2018 . So I don't think this would be anything that anyone would've thought of as artificial intelligence, but it was an algorithm and it was an automated process, so it allowed them to review hundreds of thousands of patient records. They changed, allegedly about a half a million patient records, including about a hundred thousand for this hardening of the artery walls issue. And Jonathan, our former colleagues, I can only imagine their eyes lit up when they saw , uh, a combination of, you know, something a little bit on the line, but on a massive scale with some hints of pressure on doctors and, and financial incentives. Totally irresistible. I, it's no surprise to me that the government intervened, but all of that I think is a pattern that we'll see repeated. Um, it with ai, right? Someone's gonna be using an even more sophisticated tool to do something kind of like this.
Speaker 5:Yeah, I totally agree. Nate thi this is so thi this is the, the big one-way look question that a bunch of , uh, payers, a bunch of Medicare advantage plans have been dealing with for the last decade. I think a lot of people did this, they used to do it with, you know, humans that would pull records and, and search for , um, diagnosis codes that they could add. And now it's developed into, into this case. But the, but the takeaway from this is that with ai, this could be so much of a bigger thing. The only difference is, you know, back in the, you know, original cases, and by the way, the ALA's Fraud and Abuse practice group published a really good article by some King and Spalding authors last year on these cases. Very i'll, I'll commend our listeners to go find that article. It's, it's excellent. Um, but you know, what's interesting is back in those old cases you had, you know, memos to these reviewers saying what they were looking for. You're supposed to add codes. You're not supposed to take away codes. If, if you find something that's in , been improperly reported when you're doing this with an algorithm, I don't know , or , or with , with AI even, I don't know how you, how you program the system, how you go and show that that what the , um, that that what the payer was doing was wrong. And so I think this is a big area , um, going forward, but it's also, it's not just to me, health plans. This also has a lot of potential for enforcement with , uh, with say, health systems. So in the same way that health systems can use AI to drive diagnosis and treatment, I wonder if you can't also program AI to say, Hey, while you're at it, look at our, you know, imaging centers. Look at our, look at our lab and see how busy they are. And if they're not busy, you know, why don't you just, if it's a questionable call about whether we need to run this, you know, get, get this test done, or this image thing, if we have capacity, let's go ahead and do it. And then I'm taking that a step further. What's stopping that same AI from , from then saying, okay, we are , um, you know, we make a lot of money on this image, so why don't we run this whenever it's, you know, questionable in terms of medical necessity. Why don't we ping the doctor and say, let's, let's run this extra test. So claims maximization is gonna be a really, really interesting thing , uh, going forward. I don't know what, you know, this, all of this is gonna be complex because I don't know how you sort of make a federal case out of AI programming, but it's gonna happen. And I'm, you know, really I'm, I , I don't know how DOJ is gonna make those cases out in a way that a jury's gonna understand them, but it's really interesting. And so , um, Nate, let's, let's sort of, let's start wrapping this up. Um, Nate, I'm curious if you'll give our listeners, you know, what to make of the current state of play when it comes to AI and healthcare and the potential for enforcement.
Speaker 3:Well , I think we, we know that , uh, something is coming, some kind of enforcement is coming. And by coincidence, actually on the morning of our panel, there was a , another panel of enforcers, and I was so curious to hear what they would've to say, because they talked about artificial intelligence, and I found what they said to be oddly reassuring because they, they are very much in the building phase. They have really no answers yet, only some guiding questions. But I think what we know on the current state of play is it's critical to remember that artificial intelligence is a little bit of a misnomer because the technology is extremely sophisticated and powerful at pattern matching, but it's not actually intelligent in the sense that it can't differentiate between , uh, you know, good judgment and bad judgment. So, to follow from, from like the claims maximization discussion, we were just having, you know, in those human cases, there's somebody who is exercising some kind of judgment, someone who can be deposed and , and whose communications can be reviewed. But with ai, that that transparency and that explainability can be pretty elusive. So I think that , uh, for , for use cases that has to be kept in mind and enterprises are gonna have to be able to show how they address that blind spot, right? What human oversight is there for the outcomes that are being generated, whether it's clinical decision support, claims maximization or , or some other aspect like , uh, setting prices maybe , right? Going back to, to what Chris was saying earlier. So a powerful tool with a blind spot. And I think at this point, before we get any hard and fast rules and statutes, I think you just need to be able to demonstrate that you're checking for that blind spot with real live human beings.
Speaker 5:Yeah, the , I I , I agree with all , all of that. Nate, you know, one , one thing that we, we haven't even talked about, and there was a panel, a different panel in Chicago at the AI conference that talked about this is the risk for , um, for, for bias to creep into ai , uh, in healthcare. I , I think in terms of immediate enforcement actions, that's probably the biggest risk because I think that's something that , um, that federal regulators really are looking at. Um, I think this happened after our conference, and so this is, you know, breaking brand new news. The , uh, the FTC announced a , a large settlement with a pharmacy chain over how this pharmacy chain was using AI to detect theft in its store in its stores, you know, plural. And so I, I think you're gonna see stuff like that where there is bias that creeps into the, the sort of limited AI that healthcare providers are gonna start to roll out. And that's where I think you'll, you'll see the first bit of action , um, is on the, you know, FTC front. I , I know that H-H-S-O-C-R and , and DOJ office of , um, uh, the, the Civil Rights Division is, is also really interested in , um, issues of bias and , um, because 'cause AI is largely a garbage in , garbage out system. And so I think there's been a lot of examples of how there has been bias that has been in healthcare for decades. And, and there the concerns now are, you know, are we just going to make this problem worse by continuing to allow bias to, to be a, you know, a fundamentally bad part of, of how healthcare works. And so that , that, that I think is, is, is another current state of play, is, you know, how is, how is bias going to , um, gonna , gonna seep in. So I'm curious, are there any sort of final recommendations that you two have for, for our listeners when it comes to AI and healthcare?
Speaker 3:Well, I'll lead off and, and , uh, I'll , I'll tee it up for Chris. So I do think that , um, that, that human oversight , uh, is, is kind of the core consideration because the, the kind of common theme for all the examples that we've talked about today, but , but also the examples that , uh, we're seeing in our, in our work is , uh, that there's just a , um, there , there's just a, a blind spot there where enforcers can't really test the AI effectively , um, without a whistleblower or an insider, but what they can do is test the training. Um, and so for example, the, the bias issue that you were talking about, Jonathan, right? You'd look at how was the AI trained and how was the output monitored? And, and that can sort of work around that black box issue in the middle. So I think that human oversight, that's gonna be the key. That's where I think enforcers will go. Uh, certainly that's where they'll go first. So that's, that's my observation, Chris , uh, your thoughts?
Speaker 4:Yeah, I mean, I , I I'm in sort of the same vein. I think, you know, transparency for the time being is one of the big things. 'cause getting that oversight requires sort of some understanding of what's happening. I think going back to that bias example, you can sort of see outcomes, at least in the biased example, and then compare and say, look, this, there's, you know, what the actual AI is predicting, but in a lot of , uh, circumstances, it's really hard, especially with pricing. And , um, you know, what treatment people should have, things like that to really understand what the model's doing. So I think, you know, part of that oversight is really trying to get as much transparency as possible. Um, 'cause as we've said, you know, it really can become a black box.
Speaker 5:The one thing that we talked about in Chicago at the conference that I, I think is interesting is, is again, with these, these chatbots, the, the patient facing , uh, programs that will, that have a lot of promise in, in areas like behavioral health, where you have a lot of patients who need access to their , uh, healthcare provider at irregular times. I think that's really promising. But the, the issue that, that I, my mind keeps coming back to is how flawed those systems are. So you, you, you know, there was a different a HLA podcast that, that talked about this a few months back, and it was , uh, air Canada. So Air Air Canada had this chat bot that its customers could chat with, and a , a customer was looking for a bereavement fair from Air Canada. And Air Canada said, yeah , it's no problem. You know, book it the normal amount and then submit your , uh, your request for a bereavement fair . And we'll, we'll , we'll write it off. That wasn't at all Air Canada's policy. A they just, it sort of made AI made up a fictitious bereavement policy and a , and Air Canada ended up being stuck with it in litigation because it a, like an agent, the AI was an agent of, of Air Canada. I don't want, I , I think for, for health systems that I talk to, I don't want our health systems to be, to be in the media in that way. Um, I think there's a lot of really bad, you know, outcomes that could happen when it comes to , um, when it comes to AI with patient facing care, you know, I don't know that that's necessarily an enforcement risk. Maybe, you know, creative prosecutors could come up with a theory where bad, bad, you know , something, some , uh, chatbot representing a health system could do something that's enforceable. But I just think that that's a, that's a recipe for , uh, a bit of a disaster. And so as people are thinking about integrating AI into, you know, into their normal processes, think, just be aware that there are AI is not nearly as bulletproof as a lot of people want it to be right now. Hopefully one day we'll get there , we're , but we're not there right now. And so that's, that's a , a big takeaway , uh, for me. So Nate, do you wanna take it from here and, and , uh, close this out?
Speaker 3:Yeah , absolutely. Thank you. Yeah, it's , uh, it's, it's, it's sort of funny when , uh, somebody gets one over on Air Canada, but it's not at all funny when it's, when it's , uh, patient safety or government enforcement or even civil liability, right? Those are all very serious issues for, for , uh, our listeners and our , and our clients. Well , I hope that the audience has been able to , uh, feel the enthusiasm that the three of us have for this topic. It genuinely is very interesting. And , uh, I wanted to thank my fellow panelists, but also thank A HLA for convening us, getting us together. Uh, it's great to meet you guys and share our observations , uh, with the A HLA community . So thanks again, and we will see you at a future conference. No doubt.
Speaker 2:Thank you for listening. If you enjoyed this episode, be sure to subscribe to a HLA, speaking of health law wherever you get your podcasts. To learn more about AHLA and the educational resources available to the health law community, visit American Health law org .