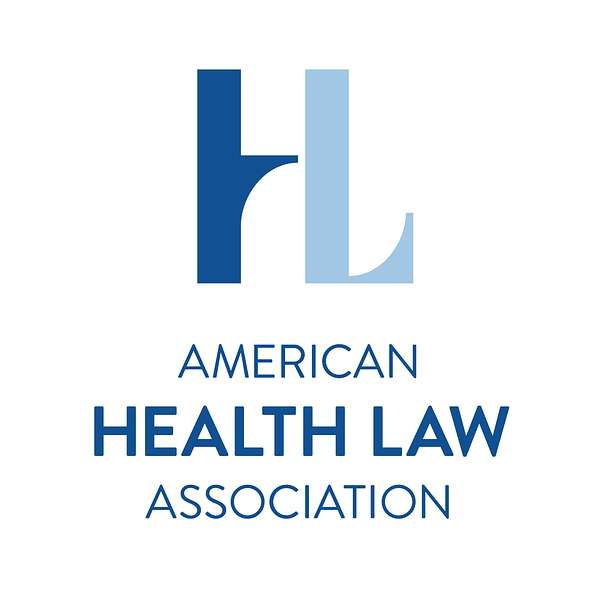
AHLA's Speaking of Health Law
The American Health Law Association (AHLA) is the largest nonprofit, nonpartisan educational organization devoted to legal issues in the health care field. AHLA's Speaking of Health Law podcasts offer thoughtful analysis and insightful commentary on the legal and policy issues affecting the American health care system.
AHLA's Speaking of Health Law
AI in Health Care: Patient Safety and Responsible Implementation
Jiayan Chen, Partner, McDermott Will & Emery, and Lauren Willens, Senior Counsel, Henry Ford Health, discuss how health care organizations can safely and responsibly implement artificial intelligence (AI). They cover the opportunities and risks of AI in health care, the current state of AI regulation, the role that patient safety organizations can play in AI safety and application, and how to apply AI in ways that enhance patient safety. Jiayan and Lauren spoke about this topic at AHLA’s 2024 Complexities of AI in Health Care in Chicago, IL.
Essential Legal Updates, Now in Audio
AHLA's popular Health Law Daily email newsletter is now a daily podcast, exclusively for AHLA Premium members. Get all your health law news from the major media outlets on this podcast! To subscribe and add this private podcast feed to your podcast app, go to americanhealthlaw.org/dailypodcast.
Stay At the Forefront of Health Legal Education
Learn more about AHLA and the educational resources available to the health law community at https://www.americanhealthlaw.org/.
<silence>
Speaker 2:This episode of A HLA speaking of health law is brought to you by A HLA members and donors like you. For more information, visit American health law.org.
Speaker 3:Hello, and thanks so much for joining us today. My name is Jayan Chen . I am a partner at the law firm of McDermott, will and Emory, where I co-lead our digital health practice and advise on all things relating to data, ai and other technologies and , uh, biomedical research , um, including in the context of , uh, compliance and strategy and, and transactions. I am delighted to be , uh, joined today by Lauren Willens of Henry Ford Health. Lauren, do you wanna introduce yourself to listeners?
Speaker 4:Sure, thanks, Diane . I am also thrilled to be here with you today. I am a senior council at Henry Ford Health, and I am our , uh, lead privacy, a EI , it , um, data privacy, HIPAA compliance , uh, expert for the system. And , uh, I, I joke that , um, I'm the lawyer you call when something nerdy happens, <laugh> 'cause it's , uh, super technical and, and I let my real healthcare colleagues deal with the , the, the , the , the hardcore patient care issues. So , uh, delighted to be here today. Great.
Speaker 3:Well, you're a good company with, with a fellow health it nerd <laugh> . Um, so on today's segment, we're gonna be talking all about , um, safety in , in health and health ai. And Lauren, you and I had the pleasure of , uh, presenting on this very topic at the inaugural A HLA Complexities of AI and Healthcare Conference in May of 2024. And we're just gonna be , uh, unpacking that in more detail on this podcast. You know, how do organizations go about responsibly implementing health ai , making sure that the AI that they're using doesn't , uh, harm patients or , or others. And also what the role is that , uh, patient safety organizations or PSOs might be able to play in AI safety. So before we get to the issue of actually how to implement AI safety programs, maybe we can start with just some table setting with respect to how can AI be deployed in healthcare? What are some of the exciting opportunities for ai? And then also what are some of the ways in which things can go awry? So, Laura , do you wanna talk through some of that?
Speaker 4:Yeah, I think it's, I think it's obvious that, you know, AI is here and it's been here actually for a while, but , um, the hot topic and the revolutionized buzz of, of health AI really, really starts with , um, the introduction of generative AI in the healthcare space. And so acknowledging that healthcare is, or AI in healthcare is here , um, we also have to take in turn that, you know, what do we do with all of that? And, and we know that it is revolutionizing healthcare, right? And so, to your point, let's start with like some of the, the exciting things that are kind of happening, which is like, we know that AI is really foundationally changing , um, the pace of which we can diagnose , um, an earlier treat disease. And so we know that we're, you know, let , do you wanna get into like some like actual examples, right? Like there's , um, there's a great AI enabled camera tool that can now be used within like radiological , um, testing like MRIs, right? Like, have you ever had an MRI , they take a really long time, or they used to take a really long time and they can be uncomfortable and loud and noisy. And so there's now technology that allows , um, for like anatomical location via AI so that you can more quicker get a patient in the right position, and then the test can go a lot quicker. So it leads to like, you know, improved satisfaction and also, you know, quicker diagnosis. Um, there's , uh, an example of early disease detection would be like a , there's a retinol image detector that can earlier diagnose diabetic retinopathy. Um, so we can prevent , um, blindness , um, earlier , um, there's increased improvement in drug development. There's administrative efficiencies. Um, clinicians can now use virtual scribing tools so that there's more one-on-one , um, patient doctor time and less time with a doctor spent, you know, typing away at the computer. Um, and then, you know, I just think that the concept of like precision medicine as a whole, you know, treating the patient disease prevention, population health , um, there's, there's so many different ways that AI can be utilized within a healthcare space. And I think, you know, to your point, we'd be remiss without talking about some of the risks, right? You know, how these tools are developed and implemented could impact patient care negatively. Um, there's a risk that the tools get it wrong. Um, there was a widely used AI tool that was , um, implemented for AI for sepsis detection, and, and it turned out the tool was really, really good at detecting patients that we already were aware were high risk, right? But it was actually only about 50 to 60% correct when it was less than clear. Um, and so that makes it more of like a coin toss diagnostic tool. And if you're relying on that, that that's pretty , um, it could be pretty challenging. Um, another example is there were some , um, the IBM Watson for healthcare. Um, it's improving, it's a currently ongoing technology, but was it , when it was originally deployed, there were some concerns across clinician , um, literature that it was giving, you know, potentially unsafe or inaccurate , um, treatment recommendations. And, and again, you know, while we're talking about this type of tool, it's not to say it's not good or it won't will be, it won't will be good in the future, but it, it , it's developing and actively ongoing. And , um, you know, I think talking about the fact that, you know, in a AI technical space, there's always a risk of, of infiltration and deep fakes , um, where whereby, you know, you have a, a third party actor who is manipulating, you know, radiological images to either include disease or remove disease. Um, and so, so that is a risk. Um, and then the last, and I think, you know, one of the most important things to consider is, you know, AI tools or as good as the data that feeds them. And so there is a real risk, and we'll spend, we'll spend some time talking about this more in depth , um, about bias based on how tools are built and trained such that, you know, if an AI tool works , um, in most of the population, but 20% of the population is at risk based on , um, age, social, demographic , um, race , um, the , you know, even if the tool's really good for most of the population, if it's not good for a segment, we need to know that and make sure not to apply it to the wrong folks.
Speaker 3:Absolutely. And, and the harm can be latent too , right? It's not always going to be the case. And, and it might actually be the case where the AI specifically was trained on data that omitted race and ethnicity information , uh, you know, out of a , you know, good faith effort to try to minimize, you know, potential biases or other discriminatory impacts that might come out of the tool. But when you do that and you don't properly adjust for other , um, components of the, of the training data, you could still ultimately have a model that is biased or, or could have , um, you know , outcomes and, and outputs that lead to discriminatory outcomes or impact . So it's really important to make sure that, for developers that are trying to figure out, you know, what data do I use to train it? And, and to constantly improve and, and refine that model , uh, how to properly calibrate the various pieces of information that, that you're using, and to be mindful of , um, you know, model drift and, you know, sort of incremental changes and, and harms that can occur over time, right?
Speaker 4:Yeah, absolutely. And I think we'll talk a little bit more about that in a little bit in terms of like, the things that need to be incorporated in the tool to reach safety , um, and, and, and be equitable, right? So, so there's, there's a lot of considerations that go into that without just talking about like the, the technical applications. I think the other things that we need to just, you know, briefly level set on as, as we're going into this topic is, and we're gonna spend a lot more time talking about some of these things, but like, the speed of innovation is really outpacing regulation, right? And so we'll talk more about that. Um, and I also think there's, you know, while we're talking about ethics and, and bias , um, there's a human trust component, right? You know, we have self-driving cars now, but not everybody's utilizing self-drive driving cars. 'cause society's not necessarily totally ready for that. Um, and we wanna make sure that that trust goes in line with, you know, none of these tools should be developed or used in isolation, right? These should be complimentary to clinical , um, oversight and guidance, whether that be from a physician or an advanced practice professional, or a , um, you know, even from just an administrative , um, oversight, you know, if you're using it for patient scheduling or access, right? And so, so those are important characteristics. And then , then I think, you know, the key legal risks that undermine or underpin all of, all of these uses and potential risks and benefits are, you know, patient privacy , um, data privacy, cybersecurity , um, have to underpin everything we're talking about.
Speaker 3:Yeah. And , and I think , um, going, going along those lines, inability or, or sort of lack of attention to transparency and, and notice to individuals who might be impacted by the use of the AI tool, right? I think when we talk about safety and , and ai, that is , um, that tends to, you know, invoke a , a specific idea in people like, oh, it's, you know, an AI tool that recommended the wrong drug or, or dose to a patient. But it can be so much broader than that , um, just in terms of like consumer patient expectations about how , uh, they're receiving care from their healthcare provider, or not being aware of the fact that there is an AI tool sitting in the room listening <laugh> to, you know, my conversation with my doctor. And so , um, I think harm in, in the context of health AI takes on a number of different facets. And that's something that, you know, we'll, we'll keep in mind as we talk through the, the rest of this , uh, this agenda today.
Speaker 4:Absolutely. And I think, you know, kind of what you're getting towards is , um, you know, and we think a lot about this from a , the , the provider side of our organization, which is, you know, what really truly is informed consent. And as we're talking about AI in all aspects, whether it's just as simple, again, as like getting into your doctor or , um, a provider thinking about how AI uses, how, how AI could be applied to how you use staffing in, in a hospital, right? All of those things have to take, you know, consent and transparency in mind. Otherwise there , you know, how do you measure efficacy, right? Efficacy can't be just measured based on improved health outcomes and reduce , um, risk. It has to be also measured against the things that we're talking about. So I think with that in mind, it probably makes sense to move to, since we're in a law podcast, right? <laugh> , Let's talk about , um, the regulatory background. So, so we know that like the EU has, has taken the lead in this aspect. And before we get to, to the executive order, which I know everybody's just dying to get to , um, I think before we even get there, be before the the EO was executed, I think it's just important to, to talk about, you know, we already have some regulatory guideposts in place , um, here in the United States, whether that be through like HIPAA privacy and security rules or FTC regulation, you know, requiring , um, that, that anything not be deceptive or biased. And, you know, dataset integrity, I think would fall under FTC authority. We also have like labor laws and unions, and many states actually have bills , um, AI bills pending. I think my last count was something around 25. But with all of that in mind, Diane , can you give us a little bit of background about the, the AI task force under HHS and the Biden executive order with respect to ai ?
Speaker 3:Yeah, definitely. So , uh, I'm sure many of those listening in are aware of the , the landmark executive order that the White House issued in late October of last year. And , um, obviously that was , uh, a much broader focus and than just health ai. But so many of the principles and mandates that were set forth in that executive order touch on , um, the topic that we're talking about today with respect to health AI safety, and so buried within the, you know, 60, 70 pages of that executive order, there is a section that , um, actually focuses on AI safety. And what it does is it requires the secretary of HHS to within a year of the, the issuance of the executive order, which is actually coming up , um, where we're talking about end of October, basically of , uh, 2024 to establish an AI safety program in collaboration with federally listed patient safety organizations, or PSOs, which we'll , uh, talk about in just a minute , um, together in consultation with the Secretaries of Defense and, and Veterans Affairs. And so what does that all you know, mean? Um, well , the executive order basically calls upon HHS to, you know , uh, put together some sort of common framework for gathering data and, and tracking issues that relate to, you know, clinical errors or other harm that could , could arise with respect to patients or even I think, you know, providers and caregivers and other folks that might be impacted by the deployment of health ai . And, and I think this is important because you can't effectively , uh, you know, do root cause analysis with respect to problems with ai, or effectively monitor and track AI and , um, uh, figure out how to , uh, remediate AI safety issues. If you don't have good data and you don't have consistent data that's across various AI tools, right? You gotta compare , um, apples to apples and oranges to oranges. And so I think the idea of figuring out some sort of common framework for , um, tracking AI safety information, there's a notion of a central tracking repository that's referenced in the executive order that's gonna be really important. Um, another thing that the executive order calls upon is , uh, for the secretary of HHS to disseminate guidance and, you know, analyses to help , uh, stakeholders with, you know, implementing best practices and , uh, other ways to , uh, remediate and, and mitigate harm and , and AI safety. And they're gonna need to work with , uh, you know, not just other regulators, but really I think AI developers, providers, patients , um, and of course, PSOs, which we'll get into in a minute. But that's, you know, really just one component of , uh, the executive order as you mentioned. There's also this HHS AI task force. There are principles that are set forth in the executive order regarding making sure that , um, you know, the AI that is disseminated is secure, that it's equitable , um, and just a lot of mandates that, you know, are at a high level spelled out in the executive order, but the devil's gonna be in the details to see how , um, you know, all the entities and , uh, you know, executive and regulatory authorities that are , um, charged with implementing the executive order. It just really remains to be seen how they're going to actually put pen to paper and implement some of the directives here around CT and, and equity. Um, the other thing that I'll mention too is that , uh, you know, given that we're well past the , uh, you know, 180 day mark , um, back in April , uh, right, right before actually our , um, a HLA AI conference, the White House had issued a 180 day update on just, you know, how things were going with respect to implementation of the executive order. And so what we know from that is that they, you know, have, at least based on that update, developed a strategy for ensuring the safety and effectiveness of AI in healthcare. And they have some, you know, frameworks apparently, that they have put together for AI testing and evaluation. Um, and they say that they've outlined some future actions for HHS to promote responsible ai uh, development and deployment. So it's, it's a very high level update. So it remains to be seen , uh, you know, what they've put together , um, by the end of October. But that's sort of all we know right now, at least in terms of , uh, implementation of that particular requirement under the executive order. But , um, you know, that's just one piece of the puzzle. Right? And Lauren , as you mentioned, there are pri , you know, existing long existing privacy and security requirements, consumer protection requirements. FDA obviously also has a big role to play with respect to the safety and efficacy of AI tools that are regulated as, you know, software as a medical device, for example, Mm-Hmm . <affirmative> , or that are used for , um, you know, clinical investigations or to support other FDA regulated activities. But , um, I also wanna touch upon , you know, one other source of legal requirements here, which is , um, you know, the, the recent final role implementing section 1557 of the Affordable Care Act. And Lauren, do you wanna kind of talk through what the mandate is in that regulation regarding monitoring for , you know, certain kinds of harms that could arise with respect to ai?
Speaker 4:Yeah. And so Section 1557 was, you know, it , it included in referenced in the eo and then , um, right around the time that we presented at A HLA , um, HHS , um, through the Office of Civil Rights, published the new final rule on, on 1557. And, and I think, you know, just to level set , you know, they publish the rule, how it's applied is really, to your point , um, to be seen, right? But, but 1557 is the, the non-discrimination provision of the Affordable Care Act that , um, prohibits discrimination on the basis of race, color, national origin, sex, age, or disability in specified health programs or activities, including those that receive , um, federal financial , um, assistance. And so the, the reg is, it , it , it makes it unlawful for healthcare providers, including doctors practices, hospitals that receive such federal assistance to refuse to treat or otherwise discriminate against , um, on an individual on the basis of one of these protected classes. And so the new final rule attempts to , um, include and reference the use of ai , um, in , in places that may implicate some of these protective classes. And so , um, HHS has actually established an AI task force to develop like a strategic plan to implement and provide guidance under the final rule. And, and the task force , uh, is looking to develop plans for AI equity, AI security, and AI oversight. And again, you know, we're still seeing how this is all playing out. Um, but things have gotten , um, even more interesting recently , um, with the recent , um, Supreme Court decision. Um, so just a couple weeks, or not even a couple weeks ago, just like, you know, right around the corner , um, the Loper Bright decision , um, really shook up , um, administrative law , um, and in abandoning the Chevron doctrine , um, after 40 years we're , we're really in healthcare, kind of like sitting on the edge of our chairs to see like, how is this all gonna play out? Um, and so the purpose of the podcast today isn't to get too deep into administrative law. Um, but just to touch, touch into it, the , the question is that we're, we're trying to understand in the healthcare regulatory and in the AI and patient safety space is how is the recent decision going to create ambiguity among these statutes and directives , um, to now that we're not deferring just to the healthcare agencies interpretations of those statutes alone. So I think we're gonna see , um, a lot of really interesting litigation and developments in this space, and it will be very interesting to see how that impacts , um, the use of AI in healthcare. But I think even more so what we're gonna see , um, from app developers and how we're gonna see app developers and software providers , um, how, how we're gonna be contracting, how we're really gonna be thinking about, and, and hopefully what that will, will lead to is some really good transparency and discussion amongst the commu the healthcare community as a whole, as to, to how to accomplish those goals in a united fashion.
Speaker 3:Absolutely. Yeah. It'll be interesting to see, you know, to what extent as a result of low bright , um, you know, both the deployers of AI that are, you know, gonna be, for example, regulated by 1557 , um, or at developers are gonna kind of take more of a wait and see approach with respect to, you know, some of the requirements that could, you know, ostensibly trickle down from, you know, overarching statutes, but, you know, don't really otherwise provide specific contours around what kinds of AI governance, for example, they need to implement. Um, and so it's, it's really, I think in the meantime, a lot of this is gonna be left up to just, you know , uh, stakeholders to negotiate amongst themselves and try to read the tea leaves as to what's ultimately going to be required , uh, of them in terms of implementing , uh, AI governance.
Speaker 4:Yeah. And I, and I think , um, you know, at our conference we had a really lively conversation regarding the concept of like, traceability, right? And so that's one that I'm, I'm really focused to see how developers and law will, will guide that. And so , um, traceability being the concept that whatever data is fed or used to develop these tools , um, the concept of traceability, which is being promoted by trade organizations such as like the a MA as well as considered under the EO and HHS guidance would require, you know, there to be , um, availability of the corresponding like metadata that is feeding and driving , um, the tools. And so , so that's one that I think will be really interesting because there are, you know, everybody shares the same goal, ideally of, you know, improved care and patient safety. Um, but, but there are other legal like IP considerations here too, right? That'll get really interesting and wonky. And so traceability is one that I think will, will be a , a hot topic and a focus topic , um, within this lo or bright kind of discussion.
Speaker 3:Absolutely.
Speaker 4:And so, so Jayne , now that we've kind of, you know, gone through like the baseline of like how we got to where we are today and where we're kind of going in the future, can you talk to us about patient safety organizations? What are they, who can participate and what are the benefits?
Speaker 3:Yeah, so I PSOs have been around for , um, decades, and I think they are going to be playing a pretty significant role with respect to AI governance and AI safety. As you know, the technology continues to evolve and, and become more ingrained within the daily, you know , um, uh, activities of, of healthcare organizations. So, just to back up a little bit, PSOs, as I mentioned, are patient safety organizations. They , um, are essentially any, you know, private or public entity. Uh , they can be affiliates or subsidiaries of an overarching , um, you know, healthcare organization or, or other legal entity. And they are, you know, the term of art is listed. Are they listed by the HHS Agency for Healthcare Research and Quality or arc as we, as we call it? And the whole idea of the PSO and the Patient Safety and Quality Improvement Act is what , what some of us call HIPAA for providers. Basically the idea is that , um, if you as a healthcare provider does a very broad term participate in A PSO and engage in patient safety activities like analyzing, you know, information about , uh, you know, best practices with respect to improving patient safety or looking at potential misdiagnoses or other things that per , perhaps went awry in connection with healthcare delivery to understand, you know , what went wrong, how can we get better , um, certain information that's generated through those patient safety activities can be , uh, uh, uh, protected by federal privilege , uh, protections attaching to that information. So , uh, with very limited exceptions that , uh, patient safety work product or PSWP as we call it, cannot be subject to, you know, a federal or state subpoena or a discovery request or, you know, otherwise admitted as evidence in connection with med mal lawsuits , um, or, or other litigation. So it's all designed to foster , uh, a culture of quality improvement and patient safety and learning. And you can see why that's got a lot of value, right? To , uh, efforts to , um, to establish AI governance and AI safety. 'cause that is also about, you know, monitoring and quality improvement and , um, you know, awareness of the potential harms that can arise in , you know, the need to, to remediate those harms. And so, PSOs, as I mentioned, you know, providers participate in PSOs, but what's interesting is that , um, as a result of the 21st Century Cares Act that was promulgated , um, or enacted a few years ago, health Information technology developers can also participate in PSOs. They're essentially treated like a provider for purposes of , uh, the p SSO statutes. And so who might be a health information technology developer that, that ostensibly could be a developer of AI tools, right? The, the term is actually not defined in, in the Cures Act. Um, so <laugh> , to your point about Loper Bright , I think there is an open question about how narrowly or broadly to interpret that term, but a more liberal interpretation could really mean anyone that's developing technology that's used in connection with , um, health or, or that's, you know , um, processing health information or perhaps facilitating the transfer or processing of health information that could include a lot of AI tools. Um, so well
Speaker 4:And couldn't that, that could, not to interrupt you, sorry to Diane in , but like theoretically to your point, which is I think an important one for our listeners, which is, you know, as a , um, in-house attorney for a provider and, you know, we have a, a , a payer, we're a payvider kind of organization, you know, that could have implications as a provider if we, you know, develop in-house or co-develop , um, AI tools or for, you know, the payer side of the organization, if there are AI tools that are used and implemented in terms of like payment and processing. So I think that that
Speaker 3:Absolutely has
Speaker 4:A very broad application, right?
Speaker 3:Absolutely. You, you, you can be a healthcare provider that is also a health information technology developer. Um, there, there's nothing at least that I have seen in the Cures Act that precludes that. So yeah, you're absolutely right. It's a very interesting framework, I think for purposes of, you know , um, leveraging an existing quality and safety concept , um, and trying to apply that to AI safety. And so, you know, understanding that this kind of information regarding patient safety, patient quality could be subject to privilege protections. Um, that, that's an interesting incentive, right? And so for , um, and , and probably of course, why the White House included PSOs as part of the, the mandate to HHS to implement some sort of AI safety program. So , uh, I , I think a lot of this remains to be seen as to, you know, how you effectively integrate PSOs into AI governance, right? Because , um, for those of you who haven't , um, read all of the pages of the Patient Safety and Quality Improvement Act and its regulations, there are a lot of details around how you set up patient safety evaluation systems. You know, how patient safety work product can be shared. Um, 'cause you can't just share it with anyone. Um, and so there's, there are details there that that needs to be figured out. But as far as, you know, then leveraging PSOs, I think a couple of things to keep in mind. One is , um, you know, even if we don't integrate a PSO into an AI governance program, you can at least, you know, include in your , um, you know, committees and, and folks that are charged with implementing AI governance folks from your PSO. These are people who, you know, think about patient safety day in and day out, right? They are great resources , um, as, as you know, organizations are trying to figure out how to , uh, develop their AI governance program. Um, and so there are a lot of concepts, I think, in the existing PSO framework that can be adapted here. And obviously the fact that health information technology developers can participate in PSOs is , um, a promising one. So let's see what comes out of the executive order. And, you know, how , uh, HHS is thinking about the role that PSOs can play. I suspect that there, you know, could, you know, be the need for some amendments to the statute to really make this all work, which, you know, let's not all hold our breath there <laugh> because we'll , uh, you know, not, not many things are are getting done on, on the Hill these days, but , um, all of this is to say that it presents some interesting opportunities and , um, I think , uh, you know, existing frameworks for AI safety.
Speaker 4:Yeah. And I, and I think that's, that's a great point because , you know, I think as we're talking, it really highlights to me like we're kind of in this really amazing but kind of uncomfortable period where innovation is, is developing and growing at the speed of light, but the reregulate regulations and, and guidance and clarity regarding, you know, who's even going to be regulated regulating this subject matter. Um, you know, it's behind and it's lagging, but like we know, we know AI is here and being used. And, and so like, I , I guess that really kind of turns us to the point in the conversation where like, let's talk about how, and I think you can really do this from, from the perspective of private practice and, you know, you represent providers, payers, you know, unique , uh, healthcare technologies. Um, I can kind of speak to this from like the, the in-house perspective, right? But like, there are a lot of considerations here knowing that AI is here and it's being used. And so like, what should we do during this really exciting but uncomfortable period to use ai , um, in a way. And today, you know, let's focus the conversation in terms of like how to set it up with really patient safety and primary focus, right? And so, you know, just to kick off my thoughts, I think this kind of like, to summarize, to give you like the preview of forthcoming attractions, right? Like, I think this is one of those things where, you know, we can't let perfect be the enemy of good, right? Like, we know that we don't have all of the answers , um, but we know that there really are like such promising and , um, amazing technology that really can improve patient safety. But we have to be uncomfortable. We have to be comfortable being uncomfortable, right?
Speaker 3:Totally. Um, as, as you know, as you mentioned, I, I kind of have the, the benefit of the outside perspective, having worked with a range of stakeholders from the developer side to the deployer side, right? Everyone is at a different , um, place in, on , on on the road, right? Um , there are some that are, are farther along in terms of developing their AI governance. Others are just getting started. Others haven't started at all. Um, we're all learning and trying to read the tea leaves in terms of what laws and regulations and standards are emerging are emerging. There's also no one size fits all . There are various, for sure different models of AI governance and, and safety programs that can be implemented. So I think , um, the key here is just to be , um, uh, you know, adaptable and to try to figure out what are some of the quick wins and ways that we can mitigate really the higher risk. Uh, you know, if, if your resources are limited and , um, you know, you need a lot of political buy-in what are some of the quick wins that we can achieve as soon as possible? And tamp down on some of the, you know, really major, you know, hotspots in terms of risks, whether it's patient safety or , um, you know, from a procurement standpoint. And, you know, trying to , uh, identify those I think is a , a good place to start.
Speaker 4:Yeah. And I, and I think the counter to like what we've just set up, which is like, you know, we know we don't know a lot of things, but like what we do know that lots of companies are doing with that fact is like, there there at least are things that we can all from whatever vantage point we're sitting. And again, I totally agree there is no one size fits all answer here, but, you know, I think it's really just important to, to recognize that the regs and the EO directly, you know, really give guidance to, there does need to be like some, if , if AI is gonna be used in connection with patient care, there needs to be some , um, governance to it. And so, you know, there needs to be the appropriate stakeholder involvement, whether that be a combination of the board key business leaders, it, legal, privacy, compliance , um, I'll even throw out IRB with respect to like, if this is, you know, clinical research that involves patients Mm-Hmm , <affirmative> , um, and, you know, maybe even some , um, entities want patient stakeholder involvement themselves, right? You know, maybe there are certain circumstances where we need the voice of patient representatives to speak , um, you know, for or against the use of certain things, even if we don't have all of the answers. And so I think it's , um, critical for, for our listeners, you know, regardless of whether you represent in-house companies or , um, you're , uh, a private practice and , um, you know, representing all sorts of industries , um, I think it's really crucial to consider, you know, a governance model that fits the needs of your organization. And so for like a provider organization where we, you know, are directly providing patient care, i , I , I think it's really, really crucial that the appropriate stakeholders have a seat at the table and that the appropriate, appropriate governance and policies and guardrails are really put in place. So not only you can , um, oversee that things are being done well, you know, you can , um, really think about as an enterprise , um, the strategy, benefits and risk of any, anything new or rolling off anything existing, right? And I think that that , um, that relationship and, and having those, those key multifaceted folks at the, at the same room or the same table, I think is really crucial to, you know, addressing patient safety and patient harm and, and patient equity. Um, and I think, you know, promoting an actively engaged group of folks really does and can very much help not just form the strategy of an organization, but um, really dial down , um, to make sure that anything that's being used or deployed really does have those key pillars in mind. So I guess what I'm trying to say is, you know, in a , a very unclear regulatory landscape , um, the onus really does become on the developers and the organizations that use and deploy these type of tools.
Speaker 3:Yeah, I, I think , um, to your point about incorporating , um, the voice of various stakeholders, and , and I think that should be done early on, right? Oh , yeah . It's not gonna help if legal or compliance just to, you know, provide an examples pulled in right before the, the tool is about to be piloted <laugh> , um, right? That all needs to be done at the outset. And, and of course, you know, not everyone needs to be at every meeting that's just going to, you know, really gum up the process with respect to , um, innovation and, you know, a quick deployment of tools that are perhaps low risk. But, you know, there does need to be some process around , uh, around i involving various stakeholders. And I think to that point, it's also not gonna help if you include someone not , um, at these committees and in these decisions if they also don't have literacy with respect to ai. So I think of course , uh, making sure that there is training and awareness and um, you know, some level of comfort and with respect to how AI works and what are some of the risks , um, that, you know, can arise with respect to the deployment of AI in healthcare for sure. And why , why this is important is, is also critical, I think, for any effective AI governance and AI safety program. So AI literacy is another thing that I would, you know, really underscore. Um, and , uh, I think the other is , uh, just being concrete and specific. So, you know, I think with a lot of the initial hand ringing around, well, what do we do? You know, AI is here and everyone's talking about it, but, you know, what do we actually do with respect to mitigating risks regarding ai? I think it's so important to actually sit down and identify for, for any given AI tool, what are the specific things that can go wrong? Um , yep . How do we measure , uh, various aspects of AI safety or efficacy? You know, what does it mean for it to work and what does it mean for it to fail ? And only when you have specific concrete examples and use cases and um, you know , uh, sort of illustrations of the harms that can arise, can you then figure out what to do about them ? So I would just, yeah , underscore Yeah,
Speaker 4:And I think that like to that, to that point, 'cause I think that's really important, Diane , is , um, to that point, like, what is success, right? Like, you know, we talked about some earlier examples of like sepsis diagnostic, and so like, you know, how do you measure success there? Like, is 86% on high risk patients enough that it's, it's useful or beneficial to employ such a sepsis tool, or is the risk that it's only gonna catch catch half? Like what is success to an organization? I don't think is, is bright line rule and what is risk to an organization is not a bright line rule, right? And so, mm-hmm , <affirmative> , I think , um, I think that's really crucial to, like, to your point of AI literacy, I think there should also be , um, some, some role-based kind of literacy, right? Like, we don't need everybody to be the experts of ai, but we need people to be the experts of AI within their appropriate domain. For instance, and I didn't reference this earlier, I should have , but like HR really deserves a seat at the table because this might not be a, you know, patient safety issue, but it might be actually, you know, a employee privacy issue, right? There's plenty of AI tools that are, you know, remote patient monitoring right now is a really hot topic. Um, and, you know, how does that implicate, you know, HR policies and things of that nature. So I think, you know, having AI literacy that's, that's role-based and, and subject matter specific is also equally as important as understanding, you know, the high level. And then, you know , to your point, really making sure that, that these discussions are not so robust that it really stifles or slows down the benefits. But the organization, I think the key here is any organization that's using ai , um, in the healthcare space and, and specifically focused to patients, really just needs to figure out a, a high level directive for the organization as well as, you know, how to deal with it in a way that , um, promotes innovation, protects patient safety and privacy, and , um, and, and really focuses on how to, how to drive the future , um, and , and make sure that it's, you know, safe, effective and secure. And, you know, I hate that we're getting to the end of this because this is like such a great conversation, but I think we should maybe, you know, wrap up with like, you know, my big takeaway has always been, and it's what I advise my internal business clients , um, and then I'd, I'd ask that you share yours, Anne , is, you know, my, my advice when people are like, well, what should I do? It's, you know, panic responsibility. Like, don't let perfect be the enemy of good. Um, be mindful of the limitations of AI and, you know, make sure anything you use is transparent, has a clinical oversight where, where it's involved with patient care and, you know, just be really mindful of ethics and patient privacy. Um, so, so that's kind of like my key, you know , um, what is it called? The elevator speech, like when anyone calls me, those are like the things that I like, just like scripted roll off my tongue, but, but what are your thoughts, Diane ?
Speaker 3:Yeah, I, I I think those are great. I would also add to that , um, you know, data and technology are your friends here? So, you know, using technology to , um, support your use of technology, standardize the information that you're gathering about the AI tools , um, enable and, and empower your team to, you know, audit and, you know, monitor the effectiveness and, and the potential issues that can arise with respect to your AI tools. By having good data and figuring out a way to, you know, use technology to support analytics with respect to those AI tools, I think that's also gonna be really important and can make lives a lot easier for those in , in your AI governance. Um, and then also contracting , um, oh yeah, I'm gonna , because so much of this right, is , um, up in the air from at least a legal and regulatory standpoint. Nothing stops, you know, two parties in, in the context of negotiating a , uh, license agreement for an AI tool to come up with their own , um, expectations and obligations around monitoring and remediating risks and other issues that come up with respect to the deployment of the AI tool. Those are all things that could be negotiated. And I say, you know, that right now is , um, I think a , a great forum for purposes of implementing , um, you know, ai, AI safety and, and governance as well. So I think those are probably the two top ones that I would highlight.
Speaker 4:I totally agree. And as , and you know, from an in-house perspective, I think like that's such a crucial consideration because we really can address a lot of these issues via contract. And, and that's where I really think our , um, our private practice colleagues like yourself are so crucial. And , you know, when things get stuck, like what is market, you know, and market being a , a moving and ambiguous term. So , um, grateful to have great minds like yours , um, outside of the organization too, to just really, you know, for in-house teams to consider. Um, it can be a really powerful add to have like a, a good private practice colleague to be able to lean on to say, you know, are , are we approaching this correctly? Or how should we be thinking about this? Um, 'cause I think it's really , it's team
Speaker 3:Effort.
Speaker 4:<laugh> exactly right. And so, well, Diane , this has been so wonderful. I've loved having this conversation with you, and I know we have lots of other things that we could be talking about, but , um, glad that we were able to do this again. We had so much fun at the original AI conference. Um, so thank you so much for taking the time to chat with me today.
Speaker 3:Of course. It was my pleasure and , uh, loved revisiting this topic and just, you know, sort of , um, also elaborating on things that I think we didn't really get a chance to talk about , um, at the conference. So this was great and, and thanks so much to A HLA for having us.
Speaker 4:Thank you all for listening and thanks again. A HLA
Speaker 2:Thank you for listening. If you enjoyed this episode, be sure to subscribe to a HLA speaking of health law wherever you get your podcasts. To learn more about ah , a and the educational resources available to the health law community, visit American Health Law .