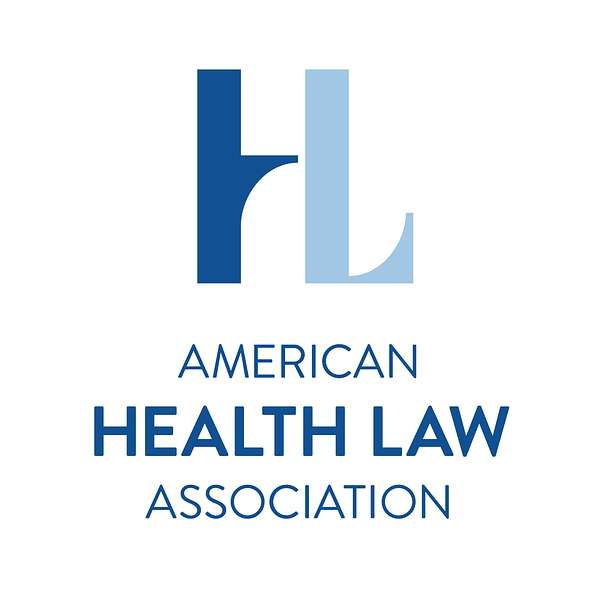
AHLA's Speaking of Health Law
The American Health Law Association (AHLA) is the largest nonprofit, nonpartisan educational organization devoted to legal issues in the health care field. AHLA's Speaking of Health Law podcasts offer thoughtful analysis and insightful commentary on the legal and policy issues affecting the American health care system.
AHLA's Speaking of Health Law
Practical Guidance to Enable Health Care Compliance Programs to Assess and Monitor AI
Andrew Mahler, Vice President of Privacy and Compliance Services, Clearwater, speaks with Kathleen Healy, Partner, Robinson Cole, and Robert Martin, Senior Legal Counsel, Mass General Brigham, about how health care compliance teams can build effective governance models, monitor legal risks, and prepare for enforcement activity related to artificial intelligence (AI). They discuss how to build an effective AI oversight framework and assess AI systems for bias and transparency, compliance considerations related to the Health Insurance Portability and Accountability Act and the 21st Century Cures Act, what federal agencies are signaling in terms of their AI priorities, and future trends shaping AI compliance in health care. Kate and Robert spoke about this topic at AHLA’s 2025 Complexities of AI in Health Care conference in Orlando, FL. Sponsored by Clearwater.
Essential Legal Updates, Now in Audio
AHLA's popular Health Law Daily email newsletter is now a daily podcast, exclusively for AHLA Premium members. Get all your health law news from the major media outlets on this podcast! To subscribe and add this private podcast feed to your podcast app, go to americanhealthlaw.org/dailypodcast.
Stay At the Forefront of Health Legal Education
Learn more about AHLA and the educational resources available to the health law community at https://www.americanhealthlaw.org/.
Support for AHLA comes from Clearwater. As the healthcare industry's largest pure-play provider of cybersecurity and compliance solutions, Clearwater helps organizations across the healthcare ecosystem move to a more secure, compliant, and resilient state so they can achieve their mission. The company provides a deep pool of experts across a broad range of cybersecurity, privacy, and compliance domains, purpose-built software that enables efficient identification and management of cybersecurity and compliance risks. and a tech-enabled 24-7-365 Security Operations Center with managed threat detection and response capabilities. For more information, visit clearwatersecurity.com.
SPEAKER_02:Good day, everyone, and welcome. This is Andrew Mahler, the Vice President of Privacy and Compliance Services at Clearwater. We're here to talk a bit more about AI. I know there's been lots of discussions, podcasts, conference panels, papers written over the past, of course, many years, but specifically in the past couple of years as AI has become a bit more ubiquitous within the healthcare space and organizations are becoming a bit more comfortable tackling AI issues. So really looking forward to the conversation today. Just taking a quick step back, as artificial intelligence becomes even more embedded in healthcare operations, and that includes, of course, clinical decision support, administrative workflows, compliance programs, as well as legal general counsel, challenge with ensuring how these tools are safe and fair and in line with existing regulations and rules, as well as a rapidly evolving legal and risk landscape. So in this episode of Speaking of Health Law, we'll explore how compliance teams can build effective governance models and monitor legal risks and prepare for the surge of emerging laws and regulations. So really excited again to have joining me with you today, Kate Healy, who's a partner with the law firm Rob Robinson Cole, and she's also the co-chair of the firm's artificial intelligence team, as well as Rob Martin, Associate General Counsel at Mass General Brigham. So just like to say hello. Welcome. Welcome to you both. And thanks for joining today. Thank you for
SPEAKER_00:having us. Great to be here with you.
SPEAKER_02:Perfect. So let's just go ahead and jump in a bit and hopefully we can make the conversation more a little fluid and tactile, and hopefully there's, I know you both will have some really interesting insights and experience to share with the listeners. So I would like to just start off by asking you a bit about AI governance. You both have spoken on this topic before and noticed that you all have spoken about the FAVES principles, so fair, appropriate, valid, effective, safe. How would you think about, or how would you advise somebody advising a healthcare organization about building a dynamic AI oversight framework? Of course, while you're helping balance robust governance, cutting edge applications, thinking about tools like ambient clinical documentation, radiology imaging.
SPEAKER_04:Andrew, I think that the first thing that I would say is Great. You should have a governance structure since that seems to be the starting point for lots of conversations. Do we need to put something in place? So I think the answer is yes for a variety of reasons. And then I think the specific... nature of the governance structure that is put in place for any particular organization really should be scaled to the size and complexity of the organization and the potential use cases for AI. Obviously, the bigger the organization, the more complicated the organization, the more complex governance structure you might want to have in place. I would also recommend that the group that comprises your governance committee, at least at the top level, multidisciplinary, right? It should have representation from the business side. In a healthcare organization, you're going to want the clinicians there. Mass General Brigham has a significant research enterprise. So you need some researchers involved. You need finance folks. You need the administrative leadership. And we've got lots and lots of capable folks on the digital team, clinicians who are also very tech savvy and researchers who are tech savvy as well. So all of those groups should be represented and then we also have um participation from clinical quality and safety folks as well so we make sure we're getting perspectives from all of those folks in addition to the compliance and legal and the risk management folks so i think that from my perspective is the starting point and then the second point the the second i think gating item would be to make sure that that group um understands that they are able to take a risk-based approach to a lot of this stuff, right? Not every use of AI is the same or requires the same level of review and analysis and tire kicking. So a risk-based framework to try to tease out high, medium, and low-risk models and the attention you need to pay to each one, I think, would be a guiding principle that probably stretches across organizations regardless of the site. And I don't know if you've got anything else to add from your experience on top of that, Kate?
SPEAKER_00:Yeah, I would really agree with that. I think the only other thing I would add is in addition to ranking them high, medium, and low in terms of their risk, I would also rank them in terms of how common they are and how critical they are to the healthcare entity at issue. So I think those are kind of the practical points to keep your eye on.
SPEAKER_02:Yeah, thank you both. I mean, I hear a lot about, you know, internal communication, you know, the importance of having good internal communication practices. You know, I think that's just really vital. And I don't know, for the two of you, I mean, I'm seeing, you know, I'm seeing more, you More of an emphasis, I think, lately when I sort of look at enforcement actions or I look at some new rules that are being discussed or set up by states or even within the federal government, I see more of an emphasis on senior leadership stakeholder involvement. So like executive involvement, the board level involvement in some of these questions. So I think some of those those thoughts really can't be underscored um just curious you know for for either of you you know anything for for you know maybe attorneys that are listening that are thinking you know well um i wonder if there's certain things you know when we're thinking about setting up a governance you know structure are there certain things we should avoid i don't know if any of you you know either of you feel comfortable talking about you know, some things you've seen or maybe just hypotheticals that you sort of worried about or kicked around that involve, you know, some lacking governance issues or some gaps that you might be willing to share to help folks, you know, think about how to avoid those things going forward. I
SPEAKER_04:think from my perspective, working in a large healthcare system, the biggest challenge challenge in that space is making sure that the various, you know, kind of AI use cases, because they're everywhere right now, right? It's not a single AI project. They are basically everything you do has some AI component now, is to make sure that folks understand that there is a process and there's a review process and a way to get it reviewed and approved. Because the biggest issue is to make sure, A, that the the business case has been vetted, right? Is the use of AI better than doing something that's an alternative or not doing it at all, right? You don't wanna do AI projects just for AI's, just for the sake of doing it. And then of course, anything in the patient care or research space, you wanna make sure that it's valid, safe and effective, a couple of the FAVES principles above. So the governance committee and any kind of operating folks under the governance committee really play a key role in making sure that that happens. Somebody should look at the model to make sure that A, it works, B, that it's safe, that it's effective, that it's sustainable. And then also once it's been implemented, you need to make sure that all of those principles continue to hold, right? Because these models change, these models drift, the use cases change, and without a robust governance function that deals with the pre-implementation, the implementation, and then the post-implementation monitoring, lots of stuff can fall through the cracks. So you want to make sure that everything is getting into the funnel at the front end so the right folks can put eyes and ears on it, and also to make sure that the post-implementation monitoring is happening so you don't find out about a quality and safety issue too late that's caused by one of these things.
SPEAKER_02:Yeah, thanks, Rob. And I mean, I think that's a perfect segue into sort of my next thought and maybe follow-up question. I noticed from the presentation you both gave earlier this year, really an emphasis on safety, bias, transparency as some critical concerns. And like you just mentioned, Rob, it's really important that there's some sort of monitoring and view into what's happening in these tools and systems to really understand whether or not these risks are present. How would you sort of, either of you, how would you think about advising internal audit compliance teams about how to effectively assess these AI systems for things like bias, accuracy, transparency?
SPEAKER_00:I can start on that one. I think there are a number of different ways that audit and compliance teams need to assess for bias accuracy and transparency. I think if a healthcare entity is involved in developing or generating datasets for the AI model, it's really important that those datasets reflect the actual patient panel, including the diversity of the healthcare entity's patient because I think if the data that's going into the model contains bias, then anything generated by the model will likely contain bias too. I think compliance teams need to try to use a variety of data sources and assess the sources accuracy and reliability. And that means including inclusion and exclusion criteria. I think compliance teams also need to talk to their vendors what criteria they use to select data sets and assess whether those criteria mitigate the risk of bias. I think compliance teams additionally need to discuss the algorithm development and validation process with their vendors. For example, does an independent team try to identify potential biases in the algorithms? How do they work with or identify the representation of minority classes? And then I think another important way to assess for bias and Transparency is by testing the tools live in the clinical environment. So that's often called sandboxing, where compliance teams might sandbox an AI model and tool and run it without it having an effect in the patient context. care model to see how it works. And then I think there's also kind of the post utilization of the AI tool and that monitoring. And there I think compliance teams can also review criteria for when and how the AI model is deployed with patients. How are the model predictions reviewed, for example? Is there a threshold data set to ensure that a model is not used to make predictions for a patient population that doesn't have a sufficient training data behind it? And then I think models need to be regularly maintained and reassessed to protect against new biases that might crop up. Rob, do you have anything else?
SPEAKER_04:No, I think that's great, Kate. I think that is a key component of certainly anything in the clinical space, right, is to make sure that your quality and safety folks have eyes on the model before it's deployed, while it's being deployed, and then afterwards to make sure that things haven't changed over time. So that's just an added piece to the normal kind of internal audit and compliance teams, I think is the clinical piece as well. But otherwise, I think. 100% agree.
SPEAKER_02:You both mentioned, you know, you sort of talked about risk framework heat maps earlier. Just curious for you both, you know, as you're thinking about, you know, auditing and monitoring of, you know, these sorts of tools and applications and systems or, you know, do you have a, I know this is maybe like a It's more like a wish list question than maybe a real question. Do you have thoughts about what systems should be looked at first in the world of AI within healthcare? Are there places that you're going to go immediately to examine AI systems versus others?
SPEAKER_00:Yeah, I can start. I mean, I think Rob hit the nail on the head early on when he said, you know, take a risk based approach. I think different entities will have, you know, different tools that are high risk. But I think starting with those high risk tools. tools is really important and ranking them so that you're clear, as Rob mentioned, the high, medium, and low risk tools. And then as I said, I think focusing on what's used most often to make sure that you've really got that covered. One of the things that I always worry about is that there are AI tools that are in use that aren't part of the inventory of AI tools. And so they slip through and nobody's really looking at them. That's what I would say. Rob?
SPEAKER_04:Yeah, I agree. I think the way that I tend to think about this is almost a you know it when you see it type of thing, which probably isn't always a great thing for a lawyer to say. But there's AI everywhere in the environment today, right? So I went to lunch and our cafeteria here uses AI. I put a salad and a Diet Pepsi down on a platform and then 10 seconds later pops up that I had a salad and Diet Pepsi and I owe$10 for lunch, right? Those types of uses of AI in the environment are really low risk and No one's going to prioritize those. For us, anything in the patient care setting that could touch patients, impact patients, have any kind of impact on the care we deliver would be on the high risk side. And then there's also high risk sort of non-patient care, but compliance issues, right? You know, billing, coding, those types of things, eligibility checks for insurance are also on the higher risk side as well.
SPEAKER_02:Makes sense. Makes sense. Yeah. Thanks for, thank you both for for sharing those thoughts. You know, I've been a part of a number of discussions over the past couple months as people are thinking about rules like the information blocking rule, 21st Century Cures Act, HIPAA, of course, and ways in which HIPAA provides certain vehicles and methods for people getting access to data and as well as required safeguards. And something that's come up, I've heard in conversations with other attorneys and compliance professionals is sort of the question of where does sort of the medical record conversation lie in the discussion of AI and maintaining data within AI systems? So I'm just curious, and this probably is a question that kind of overlaps with what I just asked, so my apologies if this is a bit duplicative, but Curious about your thoughts about these laws like 21st Century Cures Act, HIPAA, how it intersects with, you know, how they intersect with AI deployment, like chart summarization, radiology, imaging, and are there some other areas we haven't discussed where you sort of see some big compliance questions or issues around this sort of idea of AI within, you know, 21st Century Cures, HIPAA, and other similar rules? I think from
SPEAKER_04:the health system perspective, obviously HIPAA, the transparency and interoperability rules, and then lots and lots of other legal requirements have been sort of front and center for us in terms of the evaluation and the implementation of AI. Mass General Brigham was a heavy and has been a heavy adopter of the ambient clinical documentation that you mentioned earlier, and a core part of that kind of review and analysis and some of the intake and governance work was heavily focused on the privacy and security of the data that the models kind of process and also the output that's generated, kind of where is it, how is it protected, how long does it remain in those AI systems. So I think all of those legal requirements that you talked about have to be part and parcel of the governance function. On the transparency side, this hasn't been as clearly defined, I guess, globally as the HIPAA privacy and security requirements and some of the other things. But we have, as a principle, leveraged lots of the requirements that were in the 21st Century Cures Act and other things that flew down to mostly in the electronic medical record space. There were lots of requirements on those vendors about algorithms embedded in the product and transparency and sourcing of the models and how they were trained. And I think that in many respects has become a best practice even in areas that were not technically subject to that regulation. So I think it became sort of a guiding principle. The biggest issue I think on the issue of the various laws and requirements now is that folks have trouble determining exactly where things are going to go. There's been a lot of movement in the space, including lots of movement about whether AI is going to be heavily regulated or not. I think six months ago, I think folks were hoping that there might be a federal agency that might step in and bring a little bit of order to the chaos. Right now, you've got lots and lots of different entities playing in the space that makes it really hard to manage. I think that's less likely to be the case now. So it's a little bit challenging to figure out where the legal and regulatory focus is going to be, other than there seems to be a core principle to avoid any kind of regulations that would stifle innovation in the space, which obviously nobody wants to do.
SPEAKER_02:Yeah. Thanks, Rob. Really appreciate it. Again, going back and thinking about your presentation you all gave, again, I think this was maybe back in February, highlighted HHS's AI strategic plan, the FTC's focus on deceptive AI claims. I know back to your point, Rob, I mean, I think there was... Certainly a different conversation about where this was headed six, four months ago than maybe where we are now. Curious to hear from you both, though. I know this is a question that comes up a lot. How are federal agencies, whether it's HHS or the FTC, maybe even state AGs, if you have experience signaling priorities around AI compliance? And I'm assuming it's really around privacy consumer harm, but I know there's certainly other items as well.
SPEAKER_00:Yeah, I think just for historical context, I'll start out. You know, a number of the agencies really signaled priorities before the current presidential administration took office. And then when President Trump took office, he imposed via executive order a regulatory freeze. So I think, as Rob mentioned, it is somewhat hard to discern where the focus will be, at least on the federal level. I think the FTC, for example, has indicated that it's it's sorting through where the focus will be. And I think in terms of HHS, it really, it's signaled through the HHS strategic plan that it would, promote adoption of AI tools so long as they're safe, effective, and conform to ethical guidelines. And so there was that principle-based approach that was at its focus. And I think, you know, we're not sure where that will go. HHS also did publish a notice of proposed rulemaking that would, you know, strengthen cybersecurity protections around EPHI under HIPAA security rule. And I think we have read and reviewed those developments. Again, they're part of the freeze, but I would say that We did see that instead of directly regulating AI systems and tools, HHS sought comments about how to approach these new technologies. And then they also, would impose some changes under the security rule that would include things like removing the distinction between addressable and required safeguards and requiring routine review and testing of effectiveness of security measures. including encryption as a standalone technical requirement to protect data at rest and in transit. So I think those are certainly areas of focus. I expect an emphasis on data privacy and security to continue. And I think we're just waiting to see how that will emerge.
SPEAKER_02:Yeah, thanks, Kate. And I mean, I think, you know, with the, you know, we sort of had that news, I think it was earlier, maybe last week, around, you know, the House sort of moving forward to try to restrict, you know, at least advance bills that would restrict, you know, the state's ability to regulate AI. I think, I mean, I think back to, you know, to your point, there's, you know, You know, we saw a lot of signaling and it will be interesting to see how things continue to develop. you know, as we move forward this year. And I think thinking about moving forward and just trending in general, you know, curious to hear from both of you, you know, what trends are you seeing, you know, outside of anything we talked about that shape AI compliance in healthcare, you know, in the next couple of years, or are there certain things that, you know, areas where you're seeing as key battleground, you know, areas within the compliance and sort of legal framework that, you know, folks may want to be thinking about. I know you both have just a wealth of experience in this.
SPEAKER_00:I can kick it off, although I'd love to hear what Rob has to say too. I mean, I think there's gonna be increased focus on algorithmic fairness and bias. I think that there will also be added emphasis on transparency, you know, particularly with tools that, affect clinical care. So I think as patients know that AI tools are being used, they're really going to want to understand how the systems arrive at particular conclusions and become, you know, more engaged on those issues. I do think we're going to see a a continued emphasis on data privacy and security because AI involves such large amounts of data. And I think there's a lot of concern about unauthorized use and disclosure as well as really the laws being somewhat dated because AI tools can now, they have the potential to re-identify protected information in ways that the laws really didn't contemplate. I also think there's going to have to be some kind of shakeout with the patchwork of state laws that are being developed. You know, I'm I'm hopeful I'd like to see a comprehensive federal law. I think we saw with respect to confidentiality laws many, many years ago, how difficult it was to have a patchwork of state privacy laws and healthcare entities had to really navigate multiple states laws. And I think that's even more challenging in the AI context because unlike the confidentiality law context where the law of the state where the disclosure was made controlled, you have AI tools that are utilized in many different states and particularly in New England. I think it's very challenging for healthcare entities to try to comply with the whole patchwork of state laws as we see them evolve and develop. I'll pause there.
SPEAKER_02:Yeah, thanks, Kate. I don't know, Robby, any- Yeah,
SPEAKER_04:I think just two things jump out to me. The first one is I agree with Kate on the challenges that organizations face with the patchwork of state laws or even new laws that become a patchwork. Most of those regulations in the AI space seem to be focused on transparency and disclosure. And I guess one concern that I have is how useful the disclosure is or can be in all cases. We're at the point now that it is virtually impossible to receive care at a healthcare institution without AI involved in that process on the either on the administrative side or elsewhere um so if we're not careful you end up with legal requirements or obligations sort of flooding folks with information that won't really be that helpful to them right um you know if you get to the point where somebody says you know i'd like to receive care here but i don't want ai involved anywhere in my care you're not getting an mri you're not getting a ct scan or anything else which i don't think is the point of all this. The other, I think, significant legal and compliance battlegrounds going forward is going to be tied, I think, to the capabilities that certainly are there or just about there on the AI side. We're not far away from from some really, really sticky conversations about whether the AI is no longer just a tool aiding the clinicians to document clinical encounters or to provide some recommendations for them to consider. I mean, it's hard to go to a conference in this space now where somebody doesn't float the idea of AI actually treating a patient a few years down the road, right? I think somebody from CMS actually may have made that comment publicly a couple of weeks ago as well. So I think once you get close to that line, it starts to raise a whole host of issues, right? Medical device regulation, patient care, quality of care, practice of medicine stuff that I don't think anybody has their hands around yet.
SPEAKER_02:Yeah. Yeah. It's, I mean, I think there's, and I've heard some of the same comments, Rob, I think it's, you know, there's, there are a lot of really fantastic, amazing AI tools that exist. And some of the tools that exist, I mean, and you both touched on this, I mean, there's already tools that kind of stretch the imagination a bit in terms of what we can do around patient care. And then there's other things where it's a lot of, you know, wouldn't it be great if, or we're probably headed in this direction, but just don't quite know yet. So I think, I mean, this has been a fantastic conversation. I mean, I've really, really enjoyed talking with both of you. I like to leave you both with maybe any final thoughts from either of you, you know, in terms of what we talked about, or if you have, you know, sort of words to leave folks with about, you know, how people should be protecting healthcare organizations, preparing for the future. I'll just turn it back over to you for final thoughts.
SPEAKER_00:I mean, I would say one thing I would mention is just engage consistently on AI governance and really invest in a really strong AI governance program and personnel, develop those internal resources, identify external resources. I think, as we all know, AI is here to stay, and I think the regulations and laws that govern its use are emerging and are going to be here to stay as well.
SPEAKER_04:I agree with that, Kate. I think that the approach that I've landed on is that the technology is here to stay, right? It's not a question of whether or not to use it. but how best to use it. So you're not going to stop people from using it. It is everywhere. You can't pick up a phone or any other device or do just about anything without an AI component to it. So it's accepting that and figuring out the best way to utilize it in an organization, not trying to hold back the ocean and pretend it's not coming.
SPEAKER_02:All right. Well, thank you both. Kate and Rob, I think, as I said, I mean, I think this has been a, I've really learned a lot listening to both of you. Really appreciate all of your insights and the discussion. And thanks, of course, to the audience as well for listening today and hope folks found this helpful. You know, please don't hesitate to reach out if there's questions or follow-up thoughts. But otherwise, Rob, Kate, thanks again, and hope to maybe talk with you both about this sometime in the future.
SPEAKER_01:Thank you for listening. If you enjoyed this episode, be sure to subscribe to AHLA's Speaking of Health Law wherever you get your podcasts. To learn more about AHLA and the educational resources available to the health law community, visit americanhealthlaw.org.